This is the multi-page printable view of this section. Click here to print.
Docs
- 1: Chall-Manager
- 1.1: Ops Guides
- 1.1.1: Deployment
- 1.1.2: Monitoring
- 1.2: ChallMaker Guides
- 1.2.1: Create a Scenario
- 1.2.2: Software Development Kit
- 1.2.3: Update in production
- 1.2.4: Use the flag variation engine
- 1.3: Developer Guides
- 1.3.1: Extensions
- 1.3.2: Integrate with a CTF platform
- 1.4: Design
- 1.4.1: Context
- 1.4.2: The Need
- 1.4.3: Genericity
- 1.4.4: Architecture
- 1.4.5: High Availability
- 1.4.6: Hot Update
- 1.4.7: Security
- 1.4.8: Expiration
- 1.4.9: Software Development Kit
- 1.4.10: Testing
- 1.5: Tutorials
- 1.5.1: A complete example
- 1.6: Security
- 1.7: Glossary
- 2: CTFd-chall-manager
- 2.1: Get started
- 2.1.1: Setup
- 2.1.2: Create a challenge
- 2.1.3: Play the challenge
- 2.2: Guides
- 2.3: Design
1 - Chall-Manager
Warning
Currently entering public beta phase, for any issue: ctfer-io@protonmail.comChall-Manager is a MicroService whose goal is to manage challenges and their instances. Each of those instances are self-operated by the source (either team or user) which rebalance the concerns to empowers them.
It eases the job of Administrators, Operators, Chall-Makers and Players through various features, such as hot infrastructure update, non-vendor-specific API, telemetry and scalability.
Thanks to a generic Scenario concept, the Chall-Manager could be operated anywhere (e.g. a Kubernetes cluster, AWS, GCP, microVM, on-premise infrastructures) and run everything (e.g. containers, VMs, IoT, FPGA, custom resources).
1.1 - Ops Guides
1.1.1 - Deployment
You can deploy the Chall-Manager in many ways. The following table summarize the properties of each one.
Name | Maintained | Isolation | Scalable | Janitor |
---|---|---|---|---|
Kubernetes | ✅ | ✅ | ✅ | ✅ |
Binary | ⛏️ | ❌¹ | ❌ | ✅ |
Docker | ❌ | ✅ | ✅² | ✅ |
- ✅ Supported
- ❌ Unsupported
- ⛏️ Work In Progress…
¹ We do not harden the configuration in the installation script, but recommend you digging into it more as your security model requires it (especially for production purposes).
² Autoscaling is possible with an hypervisor (e.g. Docker Swarm).
Kubernetes
Note
We highly recommend the use of this deployment strategy.
We use it to test the chall-manager, and will ease parallel deployments.
This deployment strategy guarantee you a valid infrastructure regarding our functionalities and security guidelines. Moreover, if you are afraid of Pulumi you’ll have trouble creating scenarios, so it’s a good place to start !
The requirements are:
- a distributed block storage solution such as Longhorn, if you want replicas.
- an OpenTelemetry Collector, if you want telemetry data.
# Get the repository and its own Pulumi factory
git clone git@github.com:ctfer-io/chall-manager.git
cd chall-manager/deploy
# Use it straightly !
# Don't forget to configure your stack if necessary.
# Refer to Pulumi's doc if necessary.
pulumi up
Now, you’re done !
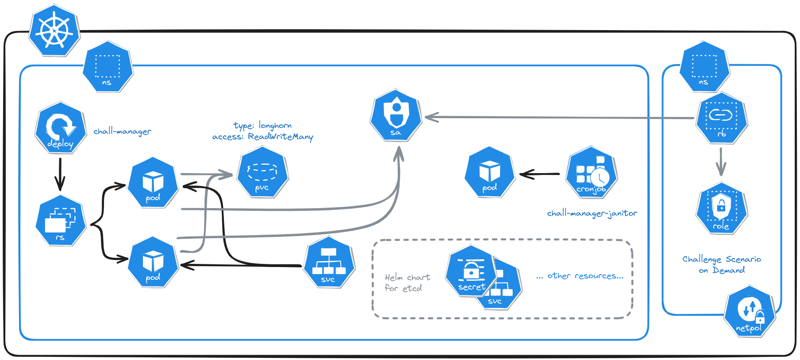
Micro Services Architecture of chall-manager deployed in a Kubernetes cluster.
Binary
Security
We highly discourage the use of this mode for production purposes, as it does not guarantee proper isolation. The chall-manager is basically a RCE-as-a-Service carrier, so if you run this on your host machine, prepare for dramatic issues.To install it on a host machine as systemd services and timers, you can run the following script.
curl -fsSL https://github.com/ctfer-io/chall-manager/blob/main/hack/setup.sh | sh
It requires:
jq
slsa-verifier
- a privileged account
Don’t forget that chall-manager requires Pulumi to be installed.
Docker
If you are unsatisfied of the way the binary install works on installation, unexisting update mecanisms or isolation, the Docker install may fit your needs.
To deploy it using Docker images, you can use the official images:
You can verify their integrity using the following commands.
slsa-verifier slsa-verifier verify-image "ctferio/chall-manager:<tag>@sha256:<digest>" \
--source-uri "github.com/ctfer-io/chall-manager" \
--source-tag "<tag>"
slsa-verifier slsa-verifier verify-image "ctferio/chall-manager-janitor:<tag>@sha256:<digest>" \
--source-uri "github.com/ctfer-io/chall-manager" \
--source-tag "<tag>"
We let the reader deploy it as (s)he needs it, but recommend you take a look at how we use systemd services and timers in the binary setup.sh
script.
Additionally, we recommend you create a specific network to isolate the docker images from other adjacent services.
For instance, the following docker-compose.yml
may fit your development needs, with the support of the janitor.
version: '3.8'
services:
chall-manager:
image: ctferio/chall-manager:v0.2.0
ports:
- "8080:8080"
chall-manager-janitor:
image: ctferio/chall-manager-janitor:v0.2.0
environment:
URL: chall-manager:8080
TICKER: 1m
1.1.2 - Monitoring
Once in production, the chall-manager provides its functionalities to the end-users.
But production can suffer from a lot of disruptions: network latencies, interruption of services, an unexpected bug, chaos engineering going a bit too far… How can we monitor the chall-manager to make sure everything goes fine ? What to monitor to quickly understand what is going on ?
Metrics
A first approach to monitor what is going on inside the chall-manager is through its metrics.
Warning
Metrics are exported by the OTLP client. If you did not configure an OTEL Collector, please refer to the deployment documentation.Name | Type | Description |
---|---|---|
challenges | int64 | The number of registered challenges. |
instances | int64 | The number of registered instances. |
You can use them to build dashboards, build KPI or anything else. They can be interesting for you to better understand the tendencies of usage of chall-manager through an event.
Tracing
A way to go deeper in understanding what is going on inside chall-manager is through tracing.
First of all, it will provide you information of latencies in the distributed locks system and Pulumi manipulations. Secondly, it will also provide you Service Performance Monitoring (SPM).
Using the OpenTelemetry Collector, you can configure it to produce RED metrics on the spans through the spanmetrics connector. When a Jaeger is bound to both the OpenTelemetry Collector and the Prometheus containing the metrics, you can monitor performances AND visualize what happens.
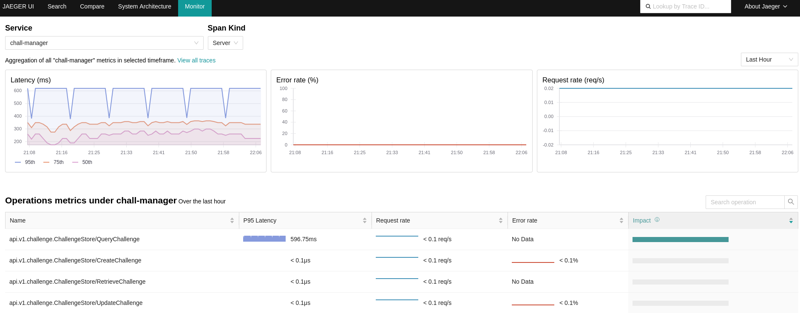
An example view of the Service Performance Monitoring in Jaeger, using the OpenTelemetry Collector and Prometheus server.
Through the use of those metrics and tracing capabilities, you could build alerts thresholds and automate responses or on-call alerts with the alertmanager.
A reference architecture to achieve this description follows.
graph TD subgraph Monitoring COLLECTOR["OpenTelemetry Collector"] PROM["Prometheus"] JAEGER["Jaeger"] ALERTMANAGER["AlertManager"] GRAFANA["Grafana"] COLLECTOR --> PROM JAEGER --> COLLECTOR JAEGER --> PROM ALERTMANAGER --> PROM GRAFANA --> PROM end subgraph Chall-Manager CM["Chall-Manager"] CMJ["Chall-Manager-Janitor"] ETCD["etcd cluster"] CMJ --> CM CM --> |OTLP| COLLECTOR CM --> ETCD end
1.2 - ChallMaker Guides
1.2.1 - Create a Scenario
You are a ChallMaker or only curious ? You want to understand how the chall-manager can spin up challenge instances on demand ? You are at the best place for it then.
This tutorial will be split up in three parts:
Design your Pulumi factory
We call a “Pulumi factory” a golang code or binary that fits the chall-manager scenario API. For details on this API, refer to the SDK documentation.
The requirements are:
Create a directory and start working in it.
mkdir my-challenge
cd $_
go mod init my-challenge
First of all, you’ll configure your Pulumi factory. The example below constitutes the minimal requirements, but you can add more configuration if necessary.
Pulumi.yaml
name: my-challenge
runtime: go
description: Some description that enable others understand my challenge scenario.
Then create your entrypoint base.
main.go
package main
import (
"github.com/pulumi/pulumi/sdk/v3/go/pulumi"
)
func main() {
pulumi.Run(func(ctx *pulumi.Context) error {
// Scenario will go there
return nil
})
}
You will need to add github.com/pulumi/pulumi/sdk/v3/go
to your dependencies: execute go mod tidy
.
Starting from here, you can get configurations, add your resources and use various providers.
For this tutorial, we will create a challenge consuming the identity from the configuration and create an Amazon S3 Bucket. At the end, we will export the connection_info
to match the SDK API.
main.go
package main
import (
"github.com/pulumi/pulumi-aws/sdk/v6/go/aws/s3"
"github.com/pulumi/pulumi/sdk/v3/go/pulumi"
"github.com/pulumi/pulumi/sdk/v3/go/pulumi/config"
)
func main() {
pulumi.Run(func(ctx *pulumi.Context) error {
// 1. Load config
cfg := config.New(ctx, "my-challenge")
config := map[string]string{
"identity": cfg.Get("identity"),
}
// 2. Create resources
_, err := s3.NewBucketV2(ctx, "example", &s3.BucketV2Args{
Bucket: pulumi.String(config["identity"]),
Tags: pulumi.StringMap{
"Name": pulumi.String("My Challenge Bucket"),
"Identity": pulumi.String(config["identity"]),
},
})
if err != nil {
return err
}
// 3. Export outputs
// This is a mockup connection info, please provide something meaningfull and executable
ctx.Export("connection_info", pulumi.String("..."))
return nil
})
}
Don’t forget to run go mod tidy
to add the required Go modules. Additionally, make sure to configure the chall-manager
pods to get access to your AWS configuration through environment variables, and add a Provider configuration in your code if necessary.
Tips & Tricks
You can compile your code to make the challenge creation/update faster, but chall-manager will automatically do it anyway to enhance performances (avoid re-downloading Go modules and Pulumi providers, and compile the scenario).
Such build could be performed through CGO_ENABLED=0 go build -o main path/to/main.go
.
Add the following configuration in your Pulumi.yaml
file to consume it, and set the binary path accordingly to the filesystem.
runtime:
name: go
options:
binary: ./main
You can test it using the Pulumi CLI with for instance the following.
pulumi stack init # answer the questions
pulumi up # preview and deploy
Make it ready for chall-manager
Now that your scenario is designed and coded accordingly to your artistic direction, you have to prepare it for the chall-manager to receive it. Make sure to remove all unnecessary files, and zip the directory it is contained within.
cd ..
zip -r my-challenge.zip ./my-challenge/*
And you’re done. Yes, it was that easy :)
But it could be even more using the SDK !
1.2.2 - Software Development Kit
When you (a ChallMaker) want to deploy a single container specific for each source, you don’t want to understand how to deploy it to a specific provider. In fact, your technical expertise does not imply you are a Cloud expert… And it was not to expect ! Writing a 500-lines long scenario fitting the API only to deploy a container is a tedious job you don’t want to do more than once: create a deployment, the service, possibly the ingress, have a configuration and secrets to handle…
For this reason, we built a Software Development Kit to ease your use of chall-manager. It contains all the features of the chall-manager without passing you the issues of API compliance.
Additionnaly, we prepared some common use-cases factory to help you focus on your CTF, not the infrastructure:
The community is free to create new pre-made recipes, and we welcome contributions to add new official ones. Please open an issue as a Request For Comments, and a Pull Request if possible to propose an implementation.
Build scenarios
Fitting the chall-manager scenario API imply inputs and outputs.
Despite it not being complex, it still requires work, and functionalities or evolutions does not guarantee you easy maintenance: offline compatibility with OCI registry, pre-configured providers, etc.
Indeed, if you are dealing with a chall-manager deployed in a Kubernetes cluster, the ...pulumi.ResourceOption
contains a pre-configured provider such that every Kubernetes resources the scenario will create, they will be deployed in the proper namespace.
Inputs
Those are fetchable from the Pulumi configuration.
Name | Required | Description |
---|---|---|
identity | ✅ | the identity of the Challenge on Demand request |
Outputs
Those should be exported from the Pulumi context.
Name | Required | Description |
---|---|---|
connection_info | ✅ | the connection information, as a string (e.g. curl http://a4...d6.my-ctf.lan ) |
flag | ❌ | the identity-specific flag the CTF platform should only validate for the given source |
Kubernetes ExposedMonopod
When you want to deploy a challenge composed of a single container, on a Kubernetes cluster, you want it to be fast and easy.
Then, the Kubernetes ExposedMonopod
fits your needs ! You can easily configure the container you are looking for and deploy it to production in the next seconds.
The following shows you how easy it is to write a scenario that creates a Deployment with a single replica of a container, exposes a port through a service, then build the ingress specific to the identity and finally provide the connection information as a curl
command.
main.go
package main
import (
"github.com/ctfer-io/chall-manager/sdk"
"github.com/ctfer-io/chall-manager/sdk/kubernetes"
"github.com/pulumi/pulumi/sdk/v3/go/pulumi"
)
func main() {
sdk.Run(func(req *sdk.Request, resp *sdk.Response, opts ...pulumi.ResourceOption) error {
cm, err := kubernetes.NewExposedMonopod(req.Ctx, &kubernetes.ExposedMonopodArgs{
Image: pulumi.String("myprofile/my-challenge:latest"),
Port: pulumi.Int(8080),
ExposeType: kubernetes.ExposeIngress,
Hostname: pulumi.String("brefctf.ctfer.io"),
Identity: pulumi.String(req.Config.Identity),
}, opts...)
if err != nil {
return err
}
resp.ConnectionInfo = pulumi.Sprintf("curl -v https://%s", cm.URL)
return nil
})
}
Requirements
To use ingresses, make sure your Kubernetes cluster can deal with them: have an ingress controller (e.g. Traefik), and DNS resolution points to the Kubernetes cluster.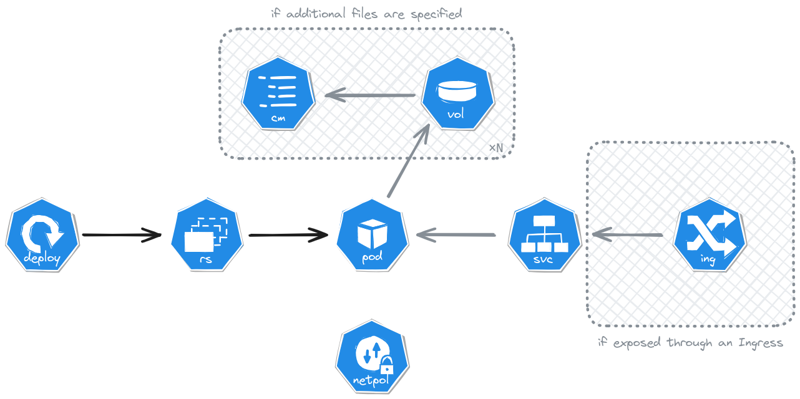
The Kubernetes ExposedMonopod architecture for deployed resources.
1.2.3 - Update in production
So you have a challenge that made its way to production, but it contains a bug or an unexpected solve ? Yes, we understand your pain: you would like to patch this but expect services interruption… It is not a problem anymore !
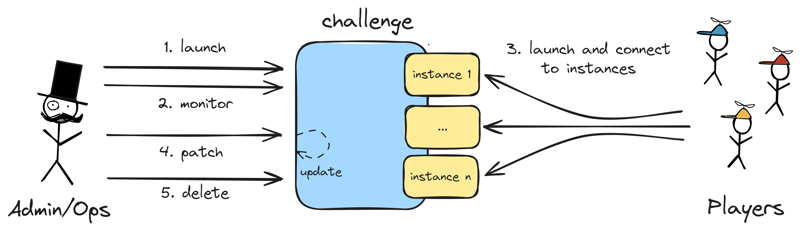
A common worklow of a challenge fix happening in production.
We adopted the reflexions of The Update Framework to provide infrastructure update mecanisms with different properties.
What to do
You will have to update the scenario, of course. Once it is fixed and validated, archive the new version.
Then, you’ll have to pick up an Update Strategy.
Update Strategy | Require Robustness¹ | Time efficiency | Cost efficiency | Availability | TL;DR; |
---|---|---|---|---|---|
Update in place | ✅ | ✅ | ✅ | ✅ | Efficient in time & cost ; require high maturity |
Blue-Green | ❌ | ✅ | ❌ | ✅ | Efficient in time ; costfull |
Recreate | ❌ | ❌ | ✅ | ❌ | Efficient in cost ; time consuming |
¹ Robustness of both the provider and resources updates. Robustness is the capability of a resource to be finely updated without re-creation.
More information on the selection of those models and how they work internally is available in the design documentation.
You’ll only have to update the challenge, specifying the Update Strategy of your choice. Chall-Manager will temporarily block operations on this challenge, and update all existing instances. This makes the process predictible and reproductible, thus you can test in a pre-production environment before production. It also avoids human errors during fix, and lower the burden at scale.
1.2.4 - Use the flag variation engine
Shareflag is considered by some as the worst part of competitions leading to unfair events, while some others consider this a strategy. We consider this a problem we could solve.
Context
In “standard” CTFs as we could most see them, it is impossible to solve this problem: if everyone has the same binary to reverse-engineer, how can you differentiate the flag per each team thus avoid shareflag ?
For this, you have to variate the flag for each source. One simple solution is to use the SDK.
Use the SDK
The SDK can variate a given input with human-readable equivalent characters in the ASCII-extended charset, making it handleable for CTF platforms (at least we expect it). If one character is out of those ASCII-character, it will be untouched.
To import this part of the SDK, execute the following.
go get github.com/ctfer-io/chall-manager/sdk
Then, in your scenario, you can create a constant that contains the “base flag” (i.e. the unvariated flag).
const flag = "my-supper-flag"
Finally, you can export the variated flag.
package main
import (
"github.com/pulumi/pulumi/sdk/v3/go/pulumi"
"github.com/ctfer-io/chall-manager/sdk"
)
const flag = "my-supper-flag"
func main() {
sdk.Run(func(req *sdk.Request, resp *sdk.Response, opts ...pulumi.ResourceOption) error {
// ...
resp.ConnectionInfo = pulumi.String("...").ToStringOutput()
resp.Flag = pulumi.Sprintf("BREFCTF{%s}", sdk.VariateFlag(req.Config.Identity, flag))
return nil
})
}
package main
import (
"github.com/pulumi/pulumi/sdk/v3/go/pulumi"
"github.com/pulumi/pulumi/sdk/v3/go/pulumi/config"
"github.com/ctfer-io/chall-manager/sdk"
)
const flag = "my-supper-flag"
func main() {
pulumi.Run(func(ctx *pulumi.Context) error {
// 1. Load config
cfg := config.New(ctx, "no-sdk")
config := map[string]string{
"identity": cfg.Get("identity"),
}
// 2. Create resources
// ...
// 3. Export outputs
ctx.Export("connection_info", pulumi.String("..."))
ctx.Export("flag", pulumi.Sprintf("BREFCTF{%s}", sdk.VariateFlag(config["identity"], flag)))
return nil
})
}
If you want to use decorator around the flag (e.g. BREFCTF{}
), don’t put it in the flag
constant else it will be variated.
1.3 - Developer Guides
1.3.1 - Extensions
How can we extend chall-manager capabilities ? You cannot, in itself: there is no support for live-mutability of functionalities, plugins, nor there will be (immutability is both an operation and security principle, determinism is a requirement).
But as chall-manager is designed as a Micro Service, you only have to reuse it !
Hacking chall-manager API
Taking a few steps back, you can abstract the chall-manager API to fit your needs:
- the
connection_info
is an Output data from the instance to the player. - the
flag
is an optional Output data from the instance to the backend. Then, if you want to pass additional data, you can use those commmunications buses.
Case studies
Follows some extension case studies.
MultiStep & MultiFlags
The original idea comes from the JointCyberRange, the following architecture is our proposal to solve the problem. In the “MultiStep challenge” problem they would like to have Jeopardy-style challenges constructed as a chain of steps, where each step has its own flag. To completly flag the challenge, the player have to get all flags in the proper order. Their target environment is an integration in CTFd as a plugin, and challenges deployed to Kubernetes.
Deploying those instances, isolating them, janitoring if necessary are requirements, thus would have been reimplemented. But chall-manager can deploy scenarios to whatever environment. Our proposal is then to cut the problem in two parts according to the Separation of Concerns Principle:
- a CTFd plugin that implement a new challenge type, and communicate with chall-manager
- chall-manager to deploy the instances
The connection_info
can be unchanged from its native usage in chall-manager.
The flag
output could contain a JSON object describing the flags chain and internal requirements.
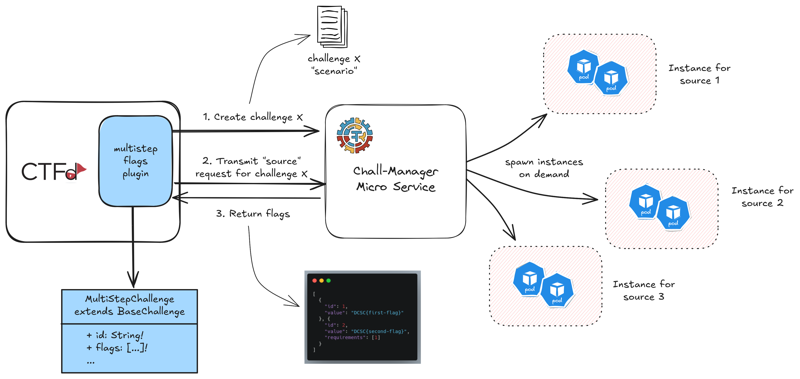
Our suggested architecture for the JCR MultiStep challenge plugin for CTFd.
Through this architecture, JCR would be able to fit their needs shortly by capitalizing on the chall-manager capabilities, thus extend its goals. Moreover, it would enable them to provide the community a CTFd plugin that does not only fit Kubernetes, thanks to the genericity.
1.3.2 - Integrate with a CTF platform
So you want to integrate chall-manager with a CTF platform ? Good job, you are contributing to the CTF ecosystem !
Here are the known integrations in CTF platforms:
The design
The API is split in two services:
- the
ChallengeStore
to handle the CRUD operations on challenges (ids, scenarios, etc.). - the
InstanceManager
to handle players CRUD operations on instances.
You’ll have to handle both services as part of the chall-manager API if you want proper integration.
We encourage you to add additional efforts around this integration, with for instance:
- management views to monitor which challenge is started for every players.
- pre-provisionning to better handle load spikes at the beginning of the event.
- add rate limiting through a mana.
- the support of OpenTelemetry for distributed tracing.
Use the proto
The chall-manager was conceived using a Model-Based Systems Engineering practice, so API models (the contracts) were written, and then the code was generated.
This makes the .proto
files the first-class citizens you may want to use in order to integrate chall-manager to a CTF platform.
Those could be found in the subdirectories here. Refer the your proto-to-code tool for generating a client from those.
If you are using Golang, you can directly use the generated clients for the ChallengeStore
and InstanceManager
services API.
If you cannot or don’t want to use the proto files, you can use the gateway API.
Use the gateway
Because some languages don’t support gRPC, or you don’t want to, you can simply communicate with chall-manager through its JSON REST API.
Chall-Manager has a gRPC+HTTP API, so you don’t have to update deployment to use it.
Nevertheless, for development purposes, we recommend you use the swagger that could be turned on with either --gw-swagger
as an arg or GATEWAY_SWAGGER=true
as a varenv.
You can then reach the Swagger at /swagger
, which should show you the following.
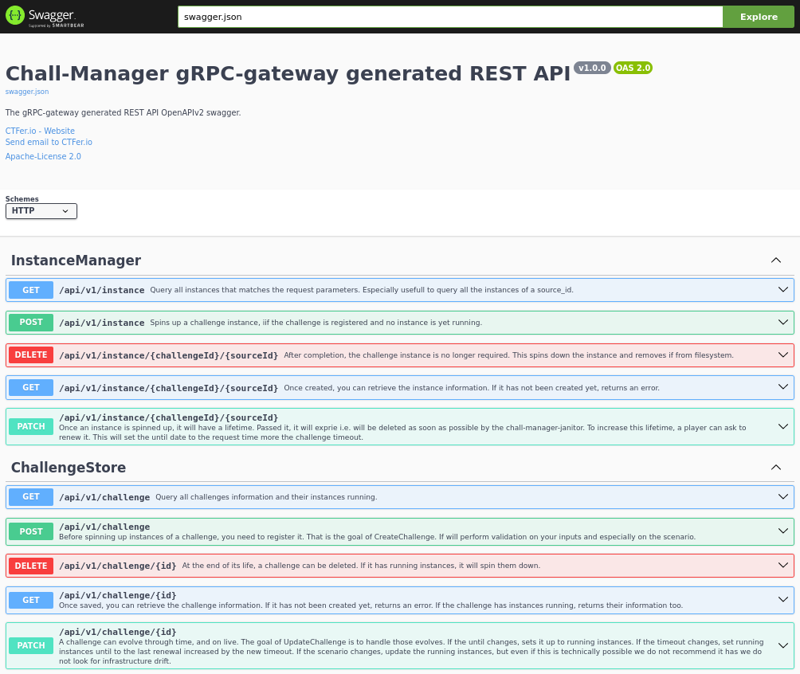
The chall-manager REST JSON API Swagger.
Use this Swagger to understand the API, and build your language-specific client in order to integrate chall-manager. We do not provide official language-specific REST JSON API clients.
1.4 - Design
In this documentation chapter, we explain what led us to design the Chall-Manager as such. We go from the CTF limitations, the Challenge on Demand problem, the functionalities, the genericity of our approach, how we made sure of its usability, to the innovative testing strategy.
1.4.1 - Context
A Capture The Flag (CTF) is an event that brings a set of players together, to challenge themselves and others on domain-specific problems. Those events could have for objective learning, or made for competing with cashprizes. They could be hold physically, virtually or hybrid.
CTFs are largely adopted in the cybersecurity community, taking place all over the world on a daily basis. In this community, plenty domains are played: web, forensic, Active Directory, cryptography, game hacking, telecommunications, steganography, mobile, OSINT, reverse engineering, programming, etc.
In general, a challenge is composed of a name, has a description, a set of hints, files and other data, shared for all. On top of those, the competition runs over points displayed on scoreboards: this is how people keep getting entertained throughout a continuous and hours-long rush. Most of the challenges find sufficient solutions to their needs with those functionalities, perhaps some does not…
If we consider other aspects of cybersecurity -as infrastructures, telecommunications, satellites, web3 and others- those solutions are not sufficient. They need specific deployments strategies, and are costfull to deploy even only once.
Nevertheless, with the emergence of the Infrastructure as Code paradigm, we think of infrastructures as reusable components, composing with pieces like for a puzzle. Technologies appeared to embrace this new paradigm, and where used by the cybersecurity community to build CTF infrastructures. Players could then share infrastructures to play those categories.
In reality, yes they could. But, de facto, they share the same infrastructure. If you are using a CTF to select the top players for a larger event, how would you be able to determine who performed better ? How do you assure their success is due to their sole effort and not a side effect of someone else work ? In the opposite direction, if you are a player who is loosing its mind on a challenge, you won’t be glad that someone broke the entire challenge thus your efforts are wortheless, isn’t it ?
What’s next ?
Read The Need to clarify the necessity of Challenge on Demand.
1.4.2 - The Need
“Sharing is caring” they said… they were wrong. Sometimes, isolating things can get spicy, but it implies replicating infrastructures. If someone soft-locks its challenge, it would be its entire problem: it won’t affect other players experience. Furthermore, you could then imagine a network challenge where your goal is to chain a vulnerability to a Man-in-the-Middle attack to get access to a router and spy on communications to steal a flag ! Or a small company infrastructure that you would have to break into and get an administrator account ! And what if the infrastructure went down ? Then it would be isolated, other players could still play in their own environment. This idea can be really productive, enhance the possibilities of a CTF and enable realistic -if not realism- attacks to take place in a controlled manner.
This is the Challenge on Demand problem: giving every player or team its own instance of a challenge, isolated, to play complex scenarios. With a solution to this problem, players and teams could use a brand new set of knowledges they could not until this: pivoting from an AWS infrastructure to an on-premise company IT system, break into a virtual bank vault to squeeze out all the belongings, hack their own Industrial Control System or IoT assets… A solution to this problem would open a myriad possibilites.
The existing solutions
As it is a widespread known limitation in the community, people tried to solve the problem. They conceived solutions that would fit their need, considering a set of functionalities and requirements, then built, released and deployed them successfully.
Some of them are famous:
- CTFd whale is a CTFd plugin able to spin up Docker containers on demand.
- CTFd owl is an alternative to CTFd whale, less famous.
- KubeCTF is another CTFd plugin made to spin up Kubernetes environments.
- Klodd is a rCTF service also made to spin up Kubernetes environments.
Nevertheless, they partially solved the root problem: those solutions solved the problem in a context (Docker, Kubernetes), with a Domain Specific Language (DSL) that does not guarantee non-vendor-lock-in nor ease use and testing, and lack functionalities such as Hot Update.
An ideal solution to this problem require:
- the use of a programmatic language, not a DSL (non-vendor-lock-in and functionalities)
- the capacity to deploy an instance without the solution itself (non-vendor-lock-in)
- the capacity to use largely-adopted technologies (e.g. Terraform, Ansible, etc., for functionalities)
- the genericity of its approach to avoid re-implementing the solution for every service provider (functionalities)
There were no existing solutions that fits those requirements… Until now.
Grey litterature survey
Follows an exhaustive grey litterature survey on the solutions made to solve the Challenge on Demand problem. To enhance this one, please open an issue or a pull request, we would be glad to improve it !
Service | CTF platform | Genericity | Technical approach | Scalable |
---|---|---|---|---|
CTFd whale | CTFd | ❌ | Docker socket | ❌¹ |
CTFd owl | CTFd | ❌ | Docker socket | ❌¹ |
KubeCTF | Agnostic, CTFd plugin | ❌ | Use Kubernetes API and annotations | ✅ |
Klodd | rCTF | ❌ | Use Kubernetes CRDs and a microservice | ✅ |
HeroCTF's deploy-dynamic | Agnostic | ❌ | API wrapper around Docker socket | ❌¹ |
4T$'s I | CTFd | ❌ | Use Kubernetes CRDs and a microservice | ✅ |
¹ not considered scalable as it reuses a Docker socket thus require a whole VM. As such a VM is not scaled automatically (despite being technically feasible), the property aligns with the limitation.
Classification for Scalable
:
- ✅ partially or completfully scalable. Classification does not goes further on criteria as the time to scale, autoscaling, load balancing, anticipated scaling, descaling, etc.
- ❌ only one instance is possible.
Litterature
More than a technical problem, the chall-manager also provides a solution to a scientific problem. In previous approaches for cybersecurity competitions, many referred to an hypothetic generic approach to the Challenge on Demand problem. None of them introduced a solution or even a realistic approach, until ours.
In those approaches to Challenge on Demand, we find:
- “PAIDEUSIS: A Remote Hybrid Cyber Range for Hardware, Network, and IoT Security Training”, Bera et al. (2021) provide Challenge on Demand for Hardware systems (Industrial Control Systems, FPGA).
- “Design of Remote Service Infrastructures for Hardware-based Capture-the-Flag Challenges”, Marongiu and Perra (2021) related to PAIDEUSIS, with the foundations for hardware-based Challenge on Demand.
- “Lowering the Barriers to Capture the Flag administration and Participation”, Kevin Chung (2017) in which it is a limitation of CTFd, where picoCTF has an “autogen” challenge feature.
- “Automatic Problem Generation for Capture-the-Flag Competitions”, Burket et al. (2015) discusses the problem of generating challenges on demand, regarding fairness evaluation for players.
- “Scalable Learning Environments for Teaching Cybersecurity Hands-on”, Vykopal et al. (2021) discusses the problem of creating cybersecurity environments, similarly to Challenge on Demand, and provide guidelines for production environments at scale.
- “GENICS: A framework for Generating Attack Scenarios for Cybersecurity Exercices on Industrial Control Systems”, Song et al. (2024) formulates a process to generate attack scenario applicable in a CTF context, technically possible through approaches like PAIDEUSIS.
Conclusions
Even if there are some solutions developed to help the community deal with the Challenge on Demand problem, we can see many limitations: only Docker and Kubernetes are covered, and none is able to provide the required genericity to consider other providers, even custom ones.
A production-ready solution would enable the community to explore new kinds of challenges, both technical and scientific.
This is why we created chall-manager: provide a free, open-source, generic, non-vendor-lock-in and ready-for-production solution. Feel free to build on top of it. Change the game.
What’s next ?
How we tackled down the complexity of building this system, starting from the architecture.
1.4.3 - Genericity
While trying to find a generic approach to deploy any infrastructure with a non-vendor-lock-in API, we looked at existing approaches. None of them proposed such an API, so we had to pave the way to a new future. But we did not know how to do it.
One day, after deploying infrastructures with Victor, we realised it was the solution. Indeed, Victor is able to deploy any Pulumi stack. This imply that the solution was already before our eyes: a Pulumi stack.
This consist the genericity layer, as easy as this.
Pulumi as the solution
To go from theory to practice, we had to make choices. One of the problem with a large genericity is it being… large, actually.
If you consider all ecosystems covered by Pulumi, to cover them you’ll require all runtimes installed on the host machine. For instance, the Pulumi Docker image is around 1.5 GB. This imply that a generic solution covering all ecosystems would be around 2 GB of memory.
Moreover, the enhancements you can propose in a language would have to be re-implemented similarly in every language, or transpiled. As transpilation is a heavy task, either manual or automatic but with a high error rate, it is not suitable for production.
Our choice was to focus on one language first (Golang), and later permit transpilation in other languages if technically automatable with a high success rate. With this choice, we would only have to deal with the Pulumi Go Docker image, around 200 MB (a 7.5 reduction factor). It could be even more reduced using minified images, using the Slim Toolkit or Chainguard Apko.
From the idea to an actual tool
With those ideas in mind, we had to transition from TRLs by implementing it in a tool. This tool could provide a service, thus the architecture was though as a Micro Service.
Doing so enable other Micro Services or CTF platforms to be developed and reuse the capabilities of chall-manager. We can then imagine plenty other challenges kind that would require Challenge on Demand:
- King of the Hill
- Attack & Defense (1 vs 1, 1 vs n, 1 vs bot)
- MultiSteps & MultiFlags (for Jeopardy)
We already plan creating 1 and 2.
What’s next
- Understand the Architecture of the microservice.
1.4.4 - Architecture
In the process of architecting a microservice, a first thing is to design the API exposed to the other microservices. Once this done, you implement it, write the underlying software that provides the service, and think of its deployment architecture to fulfill the goals.
API
We decided to avoid API conception issues by using a Model Based Systems Engineering method, to focus on the service to provide. This imply less maintenance, an improved quality and stability.
This API revolve around a simplistic class diagram as follows.
classDiagram class Challenge { + id: String! + hash: String! + timeout: Duration + until: DateTime } class Instance { + id: String! + since: DateTime! + last_renew: DateTime! + until: DateTime + connection_info: String! + flag: String } Challenge "*" *--> "1" Instance: instances
Given those two classes, we applied the Separation of Concerns Principle and split it in two cooperating services:
- the
ChallengeStore
to handle the challenges configurations - the
InstanceManager
to be the carrier of challenge instances on demand
Then, we described their objects and methods in protobuf, and using buf
we generated the Golang code for those services. The gRPC API would then be usable by any service that would want to make use of the service.
Additionally, for documentation and ease of integration, we wanted a REST JSON API: through a gateway and a swagger, that was performed still in a code-generation approach.
Software
Based on the generated code, we had to implement the functionalities to fulfill our goals.
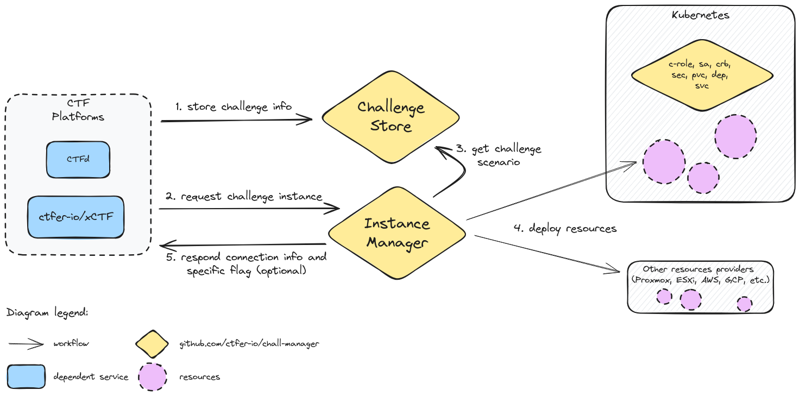
Functionalities and interactions of the chall-manager services, from a software point of view.
Nothing very special here, it was basically features implementation. This was made possible thanks to the Pulumi API that can be manipulated directly as a Go module with the auto
package.
Notice we decided to avoid depending on a file-database such as an S3-compatible (AWS S3 or MinIO) as those services may not be compatible either to offline contexts or business contexts (due to the MinIO license being GNU AGPL-v3.0).
Nevertheless, we required a solution for distributed locking systems that would not fit into chall-manager. For this, we choosed etcd. File storage replication would be handled by another solution like Longhorn or Ceph. This is further detailed in High-Availability.
In our design, we deploy an etcd instance rather than using the Kubernetes already existing one. By doing so, we avoid deep integration of our proposal into the cluster which enables multiple instances to run in parallel inside an already existing cluster. Additionnaly, it avoids innapropriate service intimacy and shared persistence issues described as good development practices in Micro Services architectures by Taibi et al. (2020) and Bogard (2017).
Deployment
Then, multiple deployment targets could be discussed. As we always think of online, offline and on-premise contexts, we wanted to be able to deploy on a non-vendor-specific hypervisor everyone would be free to use. We choosed Kubernetes.
To lift the requirements and goals of the software, we had to deploy an etcd cluster, a deployment for chall-manager and a cronjob for the janitor.
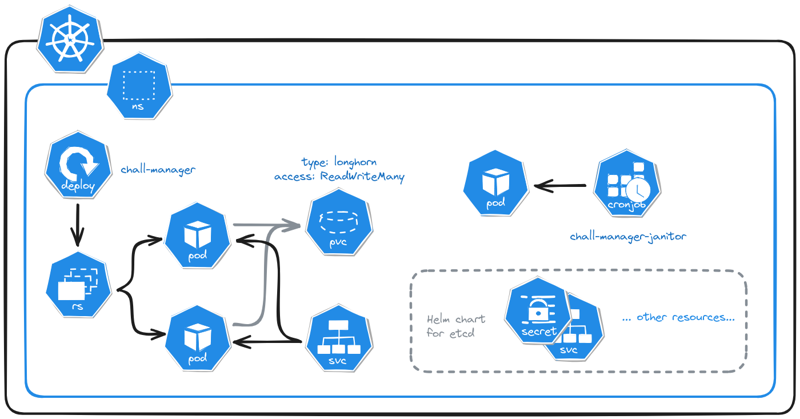
The Kubernetes deployment of a chall-manager.
One opportunity of this approach is, as chall-manager is as close as possible to the Kubernetes provider (intra-cluster), it will be easily feasible to manage resources in it. Using this, ChallMaker and Ops could deploy instances on Demand with a minimal effort. This is the reason why we recommend an alternative deployment in the Ops guides, to be able to deploy challenges in the same Kubernetes cluster.
Exposed vs. Scenario
Finally, another aspect of deployment is the exposed and scenario APIs.
The exposed one has been previously described, while the scenario one applies on scenarios once executed by the InstanceManager
.
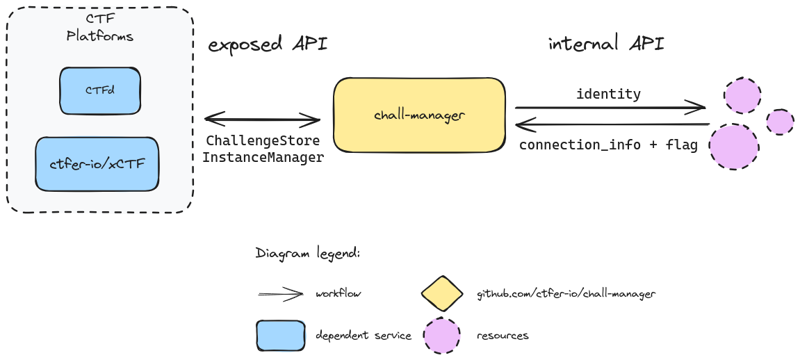
The exposed and scenario APIs.
This API will be described in the Software Development Kit.
What’s next ?
In the next section, you will understand how data consistency is performed while maintaining High Availability.
1.4.5 - High Availability
When designing an highly available application, the Availability and Consistency are often deffered to the database layer. This database explains its tradeoff(s) according to the CAP theorem. Nevertheless, chall-manager does not use a database for simplicity of use.
First of all, some definitions:
- Availability is the characteristic of an application to be reachable to the final user of services.
- High Availability contains Availability, with minimal interruptions of services and response times as low as possible.
To perform High Availability, you can use update strategies such as a Rolling Update with a maximum unavailability rate, replicate instances, etc. But it depends on a property: the application must be scalable (many instances in parallel should not overlap in their workloads if not designed so), have availability and consistency mecanisms integrated.
One question then arise: how do we assure consistency with a maximal availability ?
Fallback
As the chall-manager should scale, the locking mecanism must be distributed. In case of a network failure, it imply that whatever the final decision, the implementation should provide a recovery mecanism of the locks.
Transactions
A first reflex would be to think of transactions. They guarantee data consistency, so would be good candidates. Nevertheless, as we are not using a database that implements it, we would have to design and implement those ourselves. Such an implementation is costfull and should only happen when reusability is a key aspect. As it is not the case here (specific to chall-manager), it was a counter-argument.
Mutex
Another reflex would be to think of (distributed) mutexes. Through a mutual exclusion, we would be sure to guarantee data consistency, but nearly no availability. Indeed, a single mutex would lock out all incoming requests until the operation is completly performed. Even if it would be easy to implement, it does not match our requirements.
Such implementation would look like the following.
sequenceDiagram Upstream ->> API: Request API ->>+ Challenge: Lock Challenge ->> Challenge: Perform CRUD operation Challenge ->>- API: Unlock API ->> Upstream: Response
Multiple mutex
We need something finer than (distributed) mutex: if a challenge A is under a CRUD operation, we don’t need challenge B to not be able to handle another CRUD operation !
We can imagine one (distributed) mutex per challenge such that they won’t stuck one another. Ok, that’s fine… But what about instances ?
The same problem arise, the same solution: we can construct a chain of mutexes such that to perform a CRUD operation on an Instance
, we lock the Challenge
first, then the Instance
, unlock the Challenge
, execute the operation, and unlock the Instance
. An API call from an upstream source or service is represented with this strategy as follows.
sequenceDiagram Upstream ->> API: Request API ->>+ Challenge: Lock Challenge ->> Instance: Lock Challenge ->>- API: Unlock API ->>+ Instance: Request Instance ->> Instance: Perform CRUD operation Instance ->>- Challenge: Unlock Instance ->> API: Response API ->> Upstream: Response
One last thing, what if we want to query all challenges information (to build a dashboard, janitor outdated instances, …) ?
We would need a “Stop The World"-like mutex from which every challenge mutex would require context-relock before operation. To differenciate this from the Garbage Collector ideas, we call this a “Top-of-the-World” aka TOTW
.
The previous would now become the following.
sequenceDiagram Upstream ->> API: Request API ->>+ TOTW: Lock TOTW ->>+ Challenge: Lock TOTW ->>- API: Unlock Challenge ->> Instance: Lock Challenge ->>- TOTW: Unlock API ->>+ Instance: Request Instance ->> Instance: Perform CRUD operation Instance ->>- Challenge: Unlock Instance ->> API: Response API ->> Upstream: Response
That would guarantee consistency of the data while having availability on the API resources. Nevertheless, this availability is not high availability: we could enhance further.
Writer-Preference Reader-Writer Distributed Lock
All CRUD operations are not equal, and can be split in two groups:
- reading (Query, Read)
- writing (Create, Update, Delete).
The reading operations does not affect the state of an object, while writing ones does. Moreover, in the case of chall-manager, reading operations are nearly instantaneous and writing ones are at least 10-seconds long. How to deal with those unbalanced operations ?
As soon as discussions on OS began, researchers worked on the similar question and found solutions. They called this one the “reader-writer problem”.
In our specific case, we want writer-preference as they would largely affect the state of the resources. Using the Courtois et al. (1971) second problem solution for writer-preference reader-writer solution, we would need 5 locks and 2 counters.
For the technical implementation, we have multiple solutions: etcd, redis or valkey. We decided to choose etcd because it was already used by Kubernetes, and the etcd client v3 already implement mutex and counters.
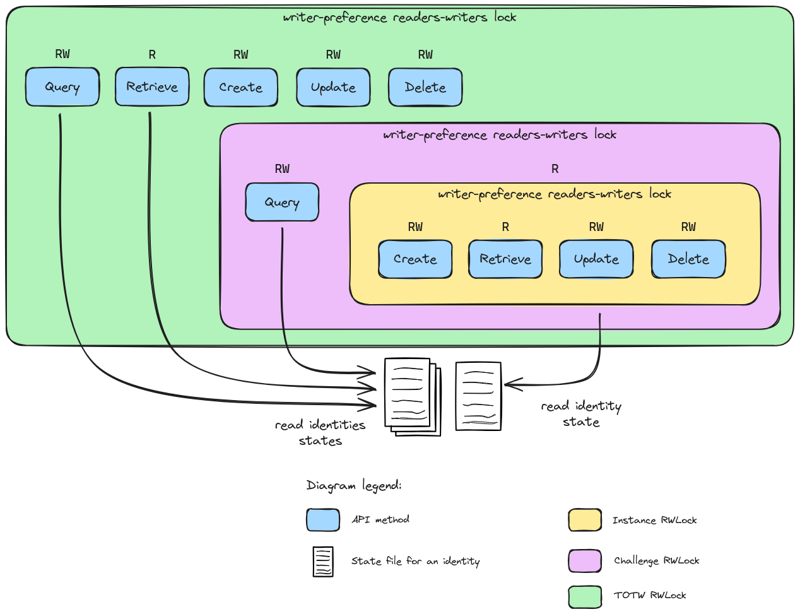
The triple chain of writer-preference reader-writer distributed locks.
With this approach, we could ensure data consistency throughout all replicas of chall-manager, and high-availability.
CRDT
Can a Conflict-Free Replicated data Type have been a solution ?
In our case, we are not looking for eventual consistency, but strict consistency. Moreover, using CRDT is costfull in development, integration and operation, so if avoidable they should be. CRDT are not the best tool to use here.
What’s next ?
Based on the guarantee of consistency and high availability, inform you on the other major problem: Hot Update.
1.4.6 - Hot Update
When a challenge is affected by a bug leading to the impossibility of solving it, or if an unexpected solve is found, you will most probably want it fixed for fairness.
If this happens on challenge with low requirements, you will fix the description, files, hints, etc. But what about challenges that require infrastructures ? You may fix the scenario, but it won’t fix the already-existing instances. If no instance has been deployed, it is fine: fixing the scenario is sufficient. Once instances has been deployed, we require a mecanism to perform this update automatically.
A reflex when dealing with a question of updates and deliveries is to refer to The Update Framework. With the embodiement of its principles, we wanted to provide macro models for hot update mecanisms. To do this, we listed a bunch of deployment strategies, analysed their inner concepts and group them on this.
Precise model | Applicable | Macro model |
---|---|---|
Blue-Green deployment | ✅ | Blue-Green |
Canary deployment | ✅ | |
Rolling deployment | ✅ | |
A/B testing | ✅ | |
Shadow deployment | ✅ | |
Red-Black deployment | ✅ | |
Highlander deployment | ✅ | Recreate |
Recreate deployment | ✅ | |
Update-in-place deployment | ✅ | Update-in-place |
Immutable infrastructure | ❌ | |
Feature toggles | ❌ | |
Dark launches | ❌ | |
Ramped deployment | ❌ | |
Serverless deployment | ❌ | |
Multi-cloud deployment | ❌ |
Strategies were classified not applicable when they did not include update mecanisms.
With the 3 macro models, we define 3 hot update strategies.
Blue-Green
The blue-green update strategy starts a new instance with the new scenario, and once it is done shuts down the old one.
It requires both instances in parallel, thus is a resources-consuming update strategy. Nevertheless, it reduces services interruptions to low or none. To the extreme, the infrastructure should be able to handle two times the instances load.
sequenceDiagram Upstream ->>+ API: Request API ->>+ New Instance: Start instance New Instance ->>- API: Instance up & running API ->>+ Old Instance: Stop instance Old Instance ->>- API: Done API ->>- Upstream: Respond new instance info
Recreate
The recreate update strategy shuts down the old one, then starts a new instance with the new scenario.
It is a resources-saving update strategy, but imply services interruptions enough time to stop the old instance and starts a new one. To the extreme, it does not require additional resources more than one time the instance load.
sequenceDiagram Upstream ->>+ API: Request API ->>+ Old Instance: Stop instance Old Instance ->>- API: Done API ->>+ New Instance: Start instance New Instance ->>- API: Instance up & running API ->>- Upstream: Respond refreshed instance info
Update-in-place
The update-in-place update strategy loads the new scenario and update resources in live.
It is a resource-saving update strategy, that imply low to none services interruptions, but require robustness in the update mecanisms. If the update mecanisms are not robust, we do not recommend this one as it could soft-lock resources in the providers. To the extreme, it does not require additional resources more than one time the instance load.
sequenceDiagram Upstream ->>+ API: Request API ->>+ Instance: Update instance Instance ->>- API: Refreshed instance API ->>- Upstream: Respond refreshed instance info
Overall
Update Strategy | Require Robustness¹ | Time efficiency | Cost efficiency | Availability | TL;DR; |
---|---|---|---|---|---|
Update in place | ✅ | ✅ | ✅ | ✅ | Efficient in time & cost ; require high maturity |
Blue-Green | ❌ | ✅ | ❌ | ✅ | Efficient in time ; costfull |
Recreate | ❌ | ❌ | ✅ | ❌ | Efficient in cost ; time consuming |
¹ Robustness of both the provider and resources updates.
What’s next ?
How did we incorporate security in such a powerfull service ? Find answers in Security.
1.4.7 - Security
The problem with the genericity of chall-manager resides in its capacity to execute any Golang code as long as it fits in a Pulumi stack i.e. anything. For this reason, there are multiple concerns to address when using the chall-manager.
Nevertheless, it also provides actionable responses to security concerns, such as shareflag and bias.
Authentication & Authorization
A question regarding such a security concern of an “RCE-as-a-Service” system is to throw authentication and authorization at it. Technically, it could fit and get justified.
Nevertheless, we think that, first of all, chall-manager replicas should not be exposed to end users and untrusted services thus Ops should put mTLS in place between trusted services and restraint communications to the bare minimum, and secondly, the Separation of Concerns Principle imply authentication and authorization are another goal thus should be achieved by another service.
Finally, authentication and authorization may but justifiable if Chall-Manager was operated as a Service. As this would not be the case with a Community Edition, we consider it out of scope.
Kubernetes
If deployed as part of a Kubernetes cluster, with a ServiceAccount
and a specific namespace to deploy instances, the chall-manager is able to mutate the architecture on the fly. To minimize the effect of such mutations, we recommend you provide this ServiceAccount
a Role
with a limited set of verbs on api groups. Those resources should only be namespaced.
To build this Role for your needs, you can use the command kubectl api-resources –-namespaced=true –o wide
to visualize a cluster resources and applicable verbs.
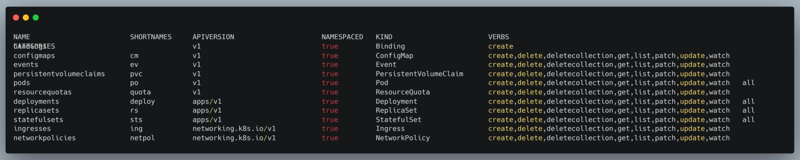
An extract of a the resources of a Kubernetes cluster and their applicable verbs.
More details on Kubernetes RBAC.
Shareflag
One of the actionable response provided by the chall-manager is through an anti-shareflag mecanism.
Each instance deployed by the chall-manager can return in its scenario a specific flag. This flag will then be used by the upstream CTF platform to ensure the source -and only it- found the solution.
Moreover, each instance-specific flag could be derived from an original constant one using the flag variation engine.
ChallOps bias
As each instance is an infrastructure in itself, variations could bias them: lighter network policies, easier brute force, etc. A scenario is not biased by essence.
If we make a risk analysis on the chall-manager capabilities and possibilities for an event, we have to consider a biased ChallMaker or Ops that produces uneven-balanced scenarios.
For this reason, the chall-manager API does not expose the source identifier of the request to the scenario code, but an identity. This is declined as follows. It strictly identifies an infrastructure identifier, the challenge the instance was requested from, and the source identifier for this instance.
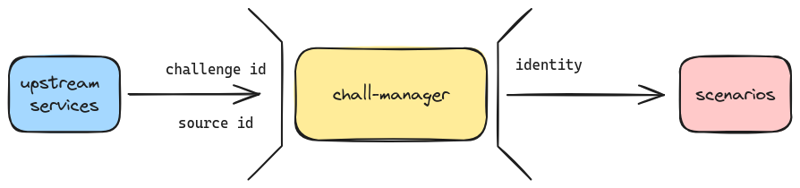
A visualization of how views are split apart to avoid the ChallOps bias.
Notice the identity is limited to 16 hexadecimals, making it compatible to multiple uses like a DNS name or a PRNG seed. This increases the possibilites of collisions, but can still cover \(16^{16} = 18.446.744.073.709.551.616\) combinations, trusted sufficient for a CTF (\(f(x,y) = x \times y - 16^{16}\), find roots: \(x \times y=16^{16} \Leftrightarrow y=\frac{16^{16}}{x}\) so roots are given by the couple \((x, \frac{16^{16}}{x})\) with \(x\) the number of challenges. With largely enough challenges e.g. 200, there is still place for \(\frac{16^{16}}{200} \simeq 9.2 \times 10^{16}\) instances each).
What’s next ?
Learn how we dealt with the resources expirations.
1.4.8 - Expiration
Context
During the CTF, we don’t want players to be capable of manipulating the infrastructure at their will: starting instances are costful, require computational capabilities, etc. It is mandatory to control this while providing the players the power to manipulate their instances at their own will.
For this reason, one goal of the chall-manager is to provide ephemeral (or not) scenarios. Ephemeral imply lifetimes, expirations and deletions.
To implement this, for each Challenge
the ChallMaker and Ops can set a timeout
in seconds after which the Instance
will be deleted once up & running, or an until
date after which the instance will be deleted whatever the timeout. When an Instance
is deployed, its start date is saved, and every update is stored for traceability. A participant (or a dependent service) can then renew an instance on demand for additional time, as long as it is under the until
date of the challenge. This is based on a hypothesis that a challenge should be solved after \(n\) minutes.
Note
The timeout should be evaluated based on expert’s point of view regarding the complexity of the conceived challenge, with a consideration of the participant skill sets (an expert can be expected to solve an introduction challenge in seconds, while a beginer can take several minutes).
There is no “rule of the thumb”, but we recommend double-testing the challenge by both a domain-expert for technical difficulty and another ChallMaker unrelated to this domain.
Deleting instances when outdated then becomes a new goal of the system, thus we cannot extend the chall-manager as it would be a rupture of the Separation of Concerns Principle: it is the goal of another service, chall-manager-janitor
. This is also justified by the frequency model applied to the janitor, which is unrelated to the chall-manager
service itself.
With such approach, other players could use the resources. Nevertheless, it requires a mecanism to wipe out infrastructure resources after a given time.
Some tools exist to do so.
Tool | Environment |
---|---|
hjacobs/kube-janitor | Kubernetes |
kubernetes-sig/boskos | Kubernetes |
rancher/aws-janitor | AWS |
Despite tools exist, they are context-specifics thus are limited: each one has its own mecanism and only 1 environment is considered. As of genericity, we want a generic approach able to handle all ecosystems without the need for specific implementations. For instance, if a ChallMaker decides to cover a unique, private and offline ecosystem, how could (s)he do ?
That is why the janitor must have the same level of genericity as chall-manager itself. Despite it is not optimal for specifics providers, we except this genericity to be a better tradeoff than covering a limited set of technologies. This modular approach enable covering new providers (vendor-specifics, public or private) without involving CTFer.io in the loop.
How it works
By using the chall-manager API, the janitor
looks up at expiration dates.
Once an instance is expired, it simply deletes it.
Using a cron, the janitor could then monitor the instances frequently.
flowchart LR subgraph Chall-Manager CM[Chall-Manager] Etcd CM --> Etcd end CMJ[Chall-Manager-Janitor] CMJ -->|gRPC| CM
If two janitors triggers in parallel, the API will maintain consistency. Errors code are to expect, but no data inconsistency.
As it does not plugs into a specific provider mecanism nor requirement, it guarantees platform agnosticity. Whatever the scenario, the chall-manager-janitor
will be able to handle it.
Follows the algorithm used to determine the instance until
date based on a challenge configuration for both until
and timeout
.
Renewing an instance re-execute this to ensure consistency with the challenge configuration.
Based on the instance until
date, the janitor will determine whether to delete it or not (\(instance.until > now() \Rightarrow delete(instance)\)).
flowchart LR Start[Compute until] Start-->until{"until == nil ?"} until---|true|timeout1{"timeout == nil ?"} timeout1---|true|out1["nil"] timeout1---|false|out2["now()+timeout"] until---|false|timeout2{"timeout == nil ?"} timeout2---|true|out3{"until"} timeout2---|false|out4{"min(now()+timeout,until)"}
What’s next ?
Listening to the community, we decided to improve further with a Software Development Kit.
1.4.9 - Software Development Kit
A first comment on chall-manager was that it required ChallMaker and Ops to be DevOps. Indeed, if we expect people to be providers’ experts to deploy a challenge, when there expertise is on a cybersecurity aspect… well, it is incoherent.
To avoid this, we took a few steps back and asked ourselves: for a beginner, what are the deployment practices that could arise form the use of chall-manager ?
A naive approach was to consider the deployment of a single Docker container in a Cloud provider (Kubernetes, GCP, AWS, etc.). For this reason, we implemented the minimal requirements to effectively deploy a Docker container in a Kubernetes cluster, exposed through an Ingress or a NodePort. The results were hundreds-line-long, so confirmed we cannot expect non-professionnals to do it.
Based on this experiment, we decided to reuse this Pulumi scenario to build a Software Development Kit to empower the ChallMaker. The references architectures contained in the SDK are available here. The rule of thumb with them is to infer the most possible things, to have a mimimum configuration for the end user.
Other features are available in the SDK.
Flag variation engine
Commonly, each challenge has its own flag. This suffers a big limitation that we can come up to: as each instance is specific to a source, we can define the flag on the fly. But this flag must not be shared with other players or it will enable shareflag.
For this reason, we provide the ability to mutate a string (expected to be the flag): for each character, if there is a variant in the ASCII-extended charset, select one of them randomly and based on the identity.
Variation rules
The variation rules follows, and if a character is not part of it, it is not mutated (each variant has its mutations evenly distributed):
a
,A
,4
,@
,ª
,À
,Á
,Â
,Ã
,Ä
,Å
,à
,á
,â
,ã
,ä
,å
b
,B
,8
,ß
c
,C
,(
,¢
,©
,Ç
,ç
d
,D
,Ð
e
,E
,€
,&
,£
,È
,É
,Ê
,Ë
,è
,é
,ê
,ë
,3
f
,F
,ƒ
g
,G
h
,H
,#
i
,I
,1
,!
,Ì
,Í
,Î
,Ï
,ì
,í
,î
,ï
j
,J
k
,K
l
,L
m
,M
n
,N
,Ñ
,ñ
o
,O
,0
,¤
,°
,º
,Ò
,Ó
,Ô
,Õ
,Ö
,Ø
,ø
,ò
,ó
,ô
,õ
,ö
,ð
p
,P
q
,Q
r
,R
,®
s
,S
,5
,$
,š
,Š
,§
t
,T
,7
,†
u
,U
,µ
,Ù
,Ú
,Û
,Ü
,ù
,ú
,û
,ü
v
,V
w
,W
x
,X
,×
y
,Y
,Ÿ
,¥
,Ý
,ý
,ÿ
z
,Z
,ž
,Ž
,
-
,_
,~
Tips & Tricks
If you want to use a decorator (e.g.BREFCTF{...}
), do not put it in the flag to variate. More info here.Limitations
We are aware that this proposition does not solve all issues: if people share their write-up, they will be able to flag. This limitation is considered out of our scope, as we don’t think the Challenge on Demand solution fits this use case.
Nevertheless, our differentiation strategy can be the basis of a proper solution to the APG-problem (Automatic Program Generation): we are able to write one scenario that will differentiate the instances per source. This could fit the input of an APG-solution.
Moreover, it considers a precise scenario of advanced malicious collaborative sources, where shareflag consider malicious collaborative sources only (more “accessible” by definition).
What’s next ?
The final step from there is to ensure the quality of our work, with testing.
1.4.10 - Testing
Generalities
In the goal of asserting the quality of a software, testing becomes a powerful tool: it provides trust in a codebase. But testing, in itself, is a whole domain of IT. Some common requirements are:
- having those tests written with a programmation language (most often the same one as the software, enable technical skills sharing among Software Engineers)
- documented (the high-level strategy should be auditable to quickly assess quality practices)
- reproducible (could be run in two distinct environments and produce the same results)
- explainable (each test case sould document its goal(s))
- systematically run, or if not possible, as frequent as possible (detect regressions as soon as possible)
To fulfill those requirements, the strategy can contain many phases which each focused on a specific aspect or condition of the software under tests.
In the following, we provide the high-level testing strategy of the chall-manager
Micro Service.
Software Engineers are invited to read it as such strategy is rare: we challenged the practices to push them beyond what the community does, with Romeo.
Testing strategy
The testing strategy contains multiple phases:
- unit tests to ensure core functions behave as expected ; those does not depend on network, os, files, etc. out thus the code and only it.
- functional tests to ensure the system behaves as expected ; those require the system to run hence a place to do so.
- integration tests to ensure the system is integrated properly given a set of targets ; those require whether the production environment or a clone of it.
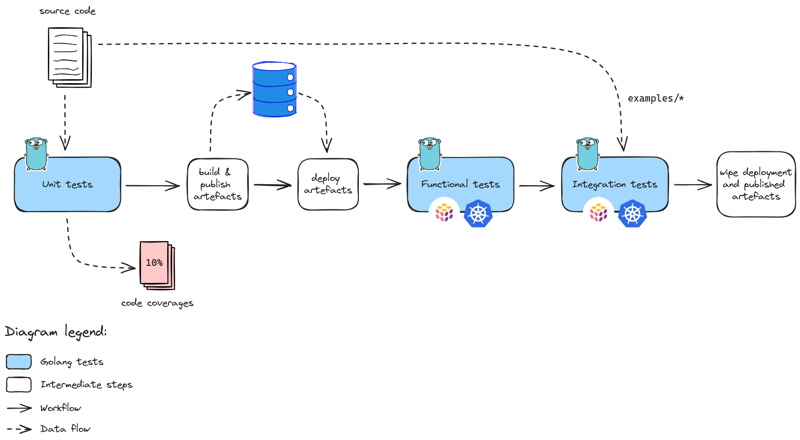
The chall-manager testing strategy.
Additional Quality Assurance steps could be found, like Stress Tests to assess Service Level Objectives under high-load conditions.
In the case of chall-manager, we write both the code and tests in Golang. To deploy infrastructures for tests, we use the Pulumi automation API and on-premise Kubernetes cluster (built using Lab and L3).
Unit tests
The unit tests revolve around the code and are isolated from anything else: no network, no files, no port listening, other tests, etc. This provides them the capacity to get run on every machine and always produce the same results: idempotence.
As chall-manager is mostly a wrapper around the Pulumi automation API to deploy scenarios, it could not be much tested using this step. Code coverage could barely reach \(10\%\) thus confidence is not sufficient.
Convention is to prefix these tests Test_U_
hence could be run using go test ./... -run=^Test_U_ -v
.
They most often have the same structure, based on Table-Driven Testing (TDT). Some diverge to fit specific needs (e.g. no regression on an issue related to a state machine).
package xxx_test
import (
"testing"
"github.com/stretchr/testify/assert"
)
func Test_U_XXX(t *testing.T) {
t.Parallel()
var tests = map[string]struct{
// ... inputs, expected outputs
}{
// ... test cases each named uniquely
// ... fuzz crashers if applicable, named "Fuzz_<id>" and a description of why it crashed
}
for testname, tt := range tests {
t.Run(testname, func(t *testing.T) {
assert := assert.New(t)
// ... the test body
// ... assert expected outputs
})
}
}
Functional tests
The functional tests revolve around the system during execution, and tests behaviors of services (e.g. state machines). Those should be reproducible, but some unexpected behaviors could arise: network interruptions, no disk available, etc. but should not be considered as the first source of a failing test (the underlying infrastructure is most often large enough to run the test, without interruption).
Convention is to prefix these tests Test_F_
hence could be run using go test ./... -run=^Test_F_ -v
.
They require a Docker image (build artifact) to be built and pushed to a registry. For Monitoring purposes, the chall-manager
binary is built with the -cover
flag to instrument the Go binary such that it exports its coverage data to filesystem.
As they require a Kubernetes cluster to run, you must define the environment variable K8S_BASE
with the DNS base URL to reach this cluster.
Cluster should fit the requirements for deployment.
Their structure depends on what needs to be tested, but follows TDT approach if applicable. Deployment is performed using the Pulumi factory.
package xxx_test
import (
"os"
"path"
"testing"
"github.com/stretchr/testify/assert"
)
func Test_F_XXX(t *testing.T) {
// ... a description of what is the goal of this test: inputs, outputs, behaviors
cwd, _ := os.Getwd()
integration.ProgramTest(t, &integration.ProgramTestOptions{
Quick: true,
SkipRefresh: true,
Dir: path.Join(cwd, ".."), // target the "deploy" directory at the root of the repository
Config: map[string]string{
// ... more configuration
},
ExtraRuntimeValidation: func(t *testing.T, stack integration.RuntimeValidationStackInfo) {
// If TDT, do it here
assert := assert.New(t)
// ... the test body
// ... assert expected outputs
},
})
}
Integration tests
The integration tests revolve around the use of the system in the production environment (or most often a clone of it, to ensure no service interruptions on the production in case of a sudden outage). In the case of chall-manager, we ensure the examples can be launched by the chall-manager. This requires us the use of multiple providers, thus a specific configuration (to sum it up, more secrets than the functional tests).
Convention is to prefix these tests Test_I_
hence could be run using go test ./... -run=^Test_I_ -v
.
They require a Docker image (build artifact) to be built and pushed to a registry. For Monitoring purposes, the chall-manager
binary is built with the -cover
flag to instrument the Go binary such that it exports its coverage data to filesystem.
As they require a Kubernetes cluster to run, you must define the environment variable K8S_BASE
with the DNS base URL to reach this cluster.
Cluster should fit the requirements for deployment.
Their structure depends on what needs to be tested, but follows TDT approach if applicable. Deployment is performed using the Pulumi factory.
package xxx_test
import (
"os"
"path"
"testing"
"github.com/stretchr/testify/assert"
)
func Test_F_XXX(t *testing.T) {
// ... a description of what is the goal of this test: inputs, outputs, behaviors
cwd, _ := os.Getwd()
integration.ProgramTest(t, &integration.ProgramTestOptions{
Quick: true,
SkipRefresh: true,
Dir: path.Join(cwd, ".."), // target the "deploy" directory at the root of the repository
Config: map[string]string{
// ... configuration
},
ExtraRuntimeValidation: func(t *testing.T, stack integration.RuntimeValidationStackInfo) {
// If TDT, do it here
assert := assert.New(t)
// ... the test body
// ... assert expected outputs
},
})
}
Monitoring coverages
Beyond testing for Quality Assurance, we also want to monitor what portion of the code is actually tested. This helps Software Development and Quality Assurance engineers to pilot where to focus the efforts, and in the case of chall-manager, what conditions where not covered at all during the whole process (e.g. an API method of a Service, or a common error).
By monitoring it and through public display, we challenge ourselves to improve to an acceptable level (e.g. \(\ge 85.00\%\)).
Disclaimer
Do not run after coverages: \(100\%\) code coverage imply no room for changes, and could be a burden to develop, run and maintain.
What you must cover are the major and minor functionalities, not all possible node in the Control Flow Graph. A good way to start this is by writing the tests by only looking at the models definition files (contracts, types, documentation).
When a Pull Request is opened (whether dependabot for automatic updates, a bot or an actual contributor), the tests are run thus helps us understand the internal changes. If the coverage decreases suddenly with the PR, reviewers would ask the PR author(s) to work on tests improvement. It also makes sure that the contribution won’t have breaking changes, thus no regressions on the covered code.
For security reasons, the tests that require platform deployments require a first review by a maintainer.
To perform monitoring of those coverages, we integrate Romeo in the testing strategy as follows.
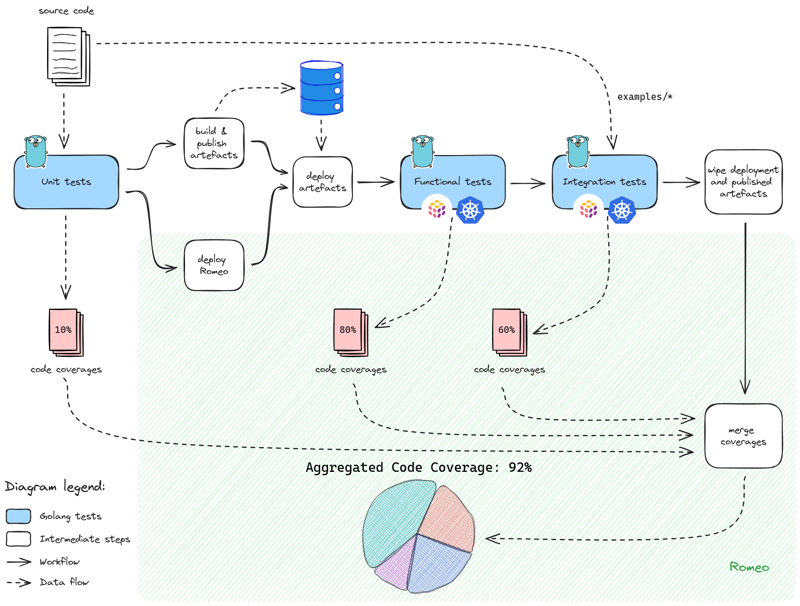
Coverages extract performed on the high-level testing strategy used for chall-manager. Values are fictive.
By combining multiple code coverages we build an aggregated code coverage higher than what standard Go tests could do.
1.5 - Tutorials
1.5.1 - A complete example
In the current documentation page, we will go through every step a ChallMaker will encounter, from the concept to players deploying instances in production.
flowchart LR Concept --> Build Build --> Scenario Scenario --> Pack Pack --> Deploy subgraph Build B1["Docker image"] end subgraph Scenario S1["Pulumi.yaml"] subgraph ExposedMonopod S2["main"] end S1 -.-> S2 end subgraph Pack P1["scenario.zip"] end
The concept
Imagine you are a ChallMaker who wants to challenge players on web application pentest.
The challenge is an application that require a licence to unlock functionalities, especially one that can read files vulnerable to local file inclusion. The initial access is due to an admin
:admin
account. The state of the application will be updated once the licence check is bypassed.
Its artistic direction is considered out of scope for now, but you’ll find this example all along our documentation !
flowchart LR A["Web app access"] A --> |"Initial access with admin:admin"| B B["Administrator account"] B --> |"License validation"| C C["Unlock vulnerable feature"] C --> |"Local File Inclusion"| D D["Read flag"]
Obviously, you don’t want players to impact others during their journey: Challenge on Demand is a solution.
Build the challenge
Using your bests software engineering skills, you conceive the application in the language of your choice, with the framework you are used to. You quickly test it, everthing behaves as expected, so you build a Write-Up for acceptance by reviewers.
This challenge is then packed into a Docker image: account/challenge:latest
We will then want to deploy this Docker image for every source that wants it.
Construct the scenario
To deploy this scenario, we don’t have big needs: one container, and a Kubernetes cluster. We’ll use the Kubernetes ExposedMonopod to ease its deployment.
First of all, we create the Pulumi.yaml
file to handle the scenario.
We write it to handle a pre-compiled binary of the scenario, for better performances.
Pulumi.yaml
name: stateful-webapp
description: The scenario to deploy the stateful web app challenge.
runtime:
name: go
options:
binary: ./main
Create the Go module using go mod init example
.
Then, we write the scenario file.
main.go
package main
import (
"github.com/ctfer-io/chall-manager/sdk"
"github.com/ctfer-io/chall-manager/sdk/kubernetes"
"github.com/pulumi/pulumi/sdk/v3/go/pulumi"
)
func main() {
sdk.Run(func(req *sdk.Request, resp *sdk.Response, opts ...pulumi.ResourceOption) error {
cm, err := kubernetes.NewExposedMonopod(req.Ctx, &kubernetes.ExposedMonopodArgs{
Image: pulumi.String("account/challenge:latest"), // challenge Docker image
Port: pulumi.Int(8080), // pod listens on port 8080
ExposeType: kubernetes.ExposeIngress, // expose the challenge through an ingress (HTTP)
Hostname: pulumi.String("brefctf.ctfer.io"), // CTF hostname
Identity: pulumi.String(req.Config.Identity), // identity will be prepended to hostname
}, opts...)
if err != nil {
return err
}
resp.ConnectionInfo = pulumi.Sprintf("curl -v https://%s", cm.URL) // a simple web server
return nil
})
}
Download the required dependencies using go mod tidy
.
To test it you can open a terminal and execute pulumi up
. It requires your host machine to have a kubeconfig
or a ServiceAccount
token in its filesystem, i.e. you are able to execute commands like kubectl get pods -A
.
Finally, compile using CGO_ENABLED=0 go build ./main main.go
.
Send it to chall-manager
The challenge is ready to be deployed. To give those information to chall-manager, you have to build the scenario zip archive.
As the scenario has been compiled, we only have to archive the Pulumi.yaml
and main
files.
zip -r scenario.zip Pulumi.yaml main
Then, you have to create the challenge (e.g. some-challenge
) in chall-manager. You can do it using the gRPC API or the HTTP gateway.
We’ll use chall-manager-cli
to do so easily.
chall-manager-cli --url chall-manager:8080 challenge create \
--id "some-challenge" \
--file scenario.zip
Now, chall-manager is able to deploy our challenge for players.
Deploy instances
To deploy instances, we’ll mock a player (e.g. mocking-bird
) Challenge on Demand request using chall-manager-cli
.
In reality, it would be to the CTF platform to handle the previous step and this one, but it is considered out of scope.
chall-manager-cli --url chall-manager:8080 instance create \
--challenge_id "some-challenge" \
--source_id "mocking-bird"
This will return us the connection information to our instance of the challenge.
1.6 - Security
RCE-as-a-Service
Through the whole documentation, we often refer to chall-manager as an RCE-as-a-Service platform. Indeed it executes scenarios on Demand, without authentication nor authorization.
For this reason, we recommend deployments to be deeply burried in the infrastructure, with firewall rules or network policies, encrypted tunnels between the dependent service(s), and anything else applicable.
Under no condition you should launch it exposed to participants and untrusted services. If not, secrets could be exfiltrated, host platform could be compromised, etc.
Kubernetes
If you are not using the recommended architecture, please make sure to not deploy instances in the same namespace as the chall-manager instances are deployed into. Elseway, players may pivot through the service and use the API for malicious purposes.
Additionally, please make sure the ServiceAccount
the chall-manager Pods
use has only its required permissions, and if possible, only on namespaced resources. To build this, you can use kubectl api-resources –-namespaced=true –o wide
.
Sharing is caring
As the chall-manager could become costful to deploy and maintain at scale, you may want to share the deployments between multiple plateforms. Notice the Community Edition does not provide isolation capabilities, so secrets, files, etc. are shared along all scenarios.
1.7 - Glossary
Challenge on Demand
The capacity of a CTF platform to empower a source to deploy its own challenges autonomously.
Scenario
It is the refinement of an artistic direction for a CTF.
In the case of Chall-Manager, it could be compared as the recipe of deployment for a given challenge. Technically, the scenario is a Pulumi entrypoint written in Go that conforms to the SDK. When launched, it deploys the source’s infrastructure and return data such as the connection information or an instance-specific flag.
Source
Either a team or user at the origin of a request. For abstraction purposes, we consider it being the same under the use of the “source” term.
Identity
An identity tie a challenge, a source and an instance request together. This last one is random (crypto) thus can’t be guessed. It enable the chall-manager to strictly identify resources as part of separate instances running at the same spot, and provide the scenario a reproductible random seed in case of update (idempotence is not guaranteed through the challenge lifecycle).
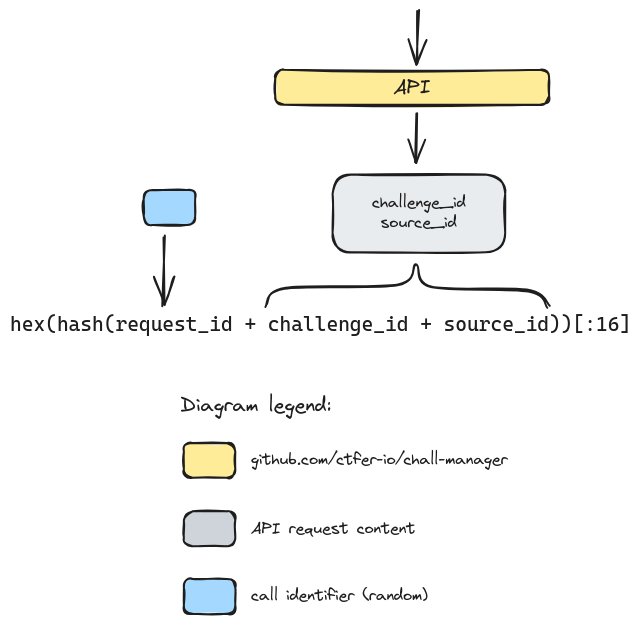
Identity production process.
Instance
An instance is the product of a scenario, once launched with an identity.
Player
A player is a CTF participant who is going to manipulate instances of challenges throughout the lifetime of the event.
ChallMaker
The designer of the challenge, often with a security expert profile on the category (s)he contributes to. This is an essential role for a CTF event, as without them, the CTF would simply not exist !
Notice it is the responsibility of the ChallMaker to make its challenge playable, not the Ops. If you can’t make your challenge run into pre-prod/prod, you can’t blame the Ops.
(S)He collaborates with plenty profiles:
- other ChallMakers to debate its ideas and assess the difficulty.
- Ops to make sure its challenges can reach production smoothly.
- Admins to discuss the technical feasibility of its challenges, for instance if it requires FPGAs, online platforms as GCP or AWS, etc. or report on the status of the CTF.
- an artistic direction, graphical designer, etc. to assist on the coherence of the challenge in the whole artistic process.
Ops
The Operator of the event who ensure the infrastructure is up and running, everything runs untroubled thus players can compete. They do not need to be security experts, but might probably be due to the community a CTF brings.
They are the rulers of the infrastructure, its architecture and its incidents. ChallMakers have both fear and admiration on them, as they enable playing complex scenarios but are one click away of destructing everything.
(S)He collaborates with various profiles:
- other Ops as a rubber ducky, a mental support during an outage or simply to work in group.
- ChallMakers to assist writing the scenarios in case of a difficulty or a specific infrastructure architecture or requirement.
- Admins to report on the current lifecycle of the infrastructures, the incidents, or provide ideas for evolutions such as a partnership.
- a technical leader to centralize the reflexions on architectures and means to enable the artistic direction achieving their goals.
Administrator
The Administrator is the showcase of the event. (S)He takes responsibility and decisions during the creation process of the event, make sure to synchronize teams throughout the development of the artistic and technical ideas, and manage partnerships if necessary. They are the managers through the whole event, before and after, not only during the CTF.
(S)He basically collaborates with everyone, which is a double-edged sword: you take the gratification of the whole effort, but have no time to rest.
2 - CTFd-chall-manager
Warning
Currently entering public beta phase, for any issue: ctfer-io@protonmail.com2.1 - Get started
2.1.1 - Setup
Goal
This tutorial will guide you through the installation and configuration of the CTFd-chall-manager plugin to use chall-manager.
Prerequisites
Ensure you have chall-manager running before starting this tutorial. You can find the relevant documentation for setup instructions.
Install the plugin
If you are not using the docker-compose.yml
file, you need to clone the repository into CTFd/CTFd/plugins/ctfd-chall-manager
.
# Clone the CTFd repository
git clone https://github.com/CTFd/CTFd
# Clone the plugin repository
git clone https://github.com/ctfer-io/ctfd-chall-manager CTFd/CTFd/plugins/ctfd-chall-manager
# (optional) Start Redis with Docker
docker run -d -p 6379:6379 redis:<version>
## (optional) Configure plugin to use redis serveur
export REDIS_URL=redis://localhost:6379
# Start CTFd
cd CTFd
python3 -m venv venv
source venv/bin/activate
pip3 install -r requirements.txt
python3 serve.py
# Clone the plugin repository
git clone https://github.com/ctfer-io/ctfd-chall-manager
# Create Docker network
docker network create testing
# (optional) Start Redis with Docker
docker run -d --name redis-svc --network testing redis:<version>
# Start CTFd with Docker
docker run -d -p 8000:8000 [-e REDIS_URL=redis://redis-svc:6379] -v ./ctfd-chall-manager:/opt/CTFd/CTFd/plugins/ctfd-chall-manager --network testing ctfd/ctfd:<version>
Tips & Tricks
You can use assets in GitHub releases instead of cloning the whole repository: https://github.com/ctfer-io/ctfd-chall-manager/releasesAfter completing this step, you should be able to access the plugin settings configuration in the CTFd UI.
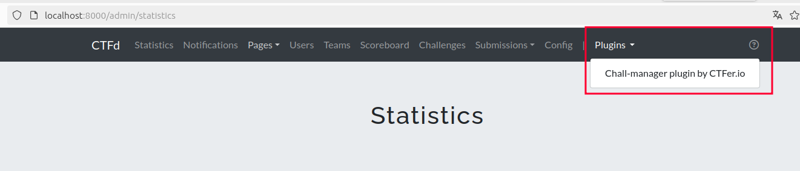
The plugin is visible in the UI
If the plugin does not appear, verify your container volume mounts, then check the CTFd logs for import module entries, such as:
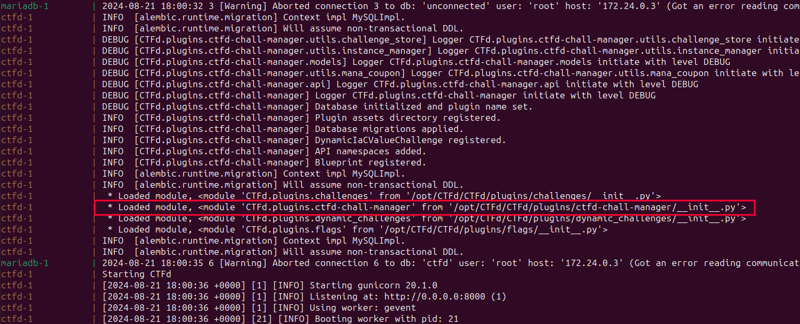
The plugin is visible in the logs
Configure the plugin to use chall-manager
To connect the plugin to chall-manager, go to the plugin settings.
The default configuration is:
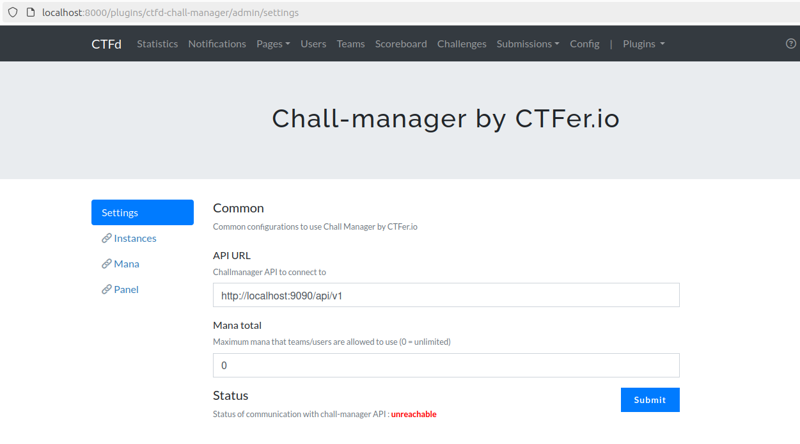
Default plugin configuration
Adjust the plugin settings to match your environment, ensuring CTFd can communicate with chall-manager. For instance:
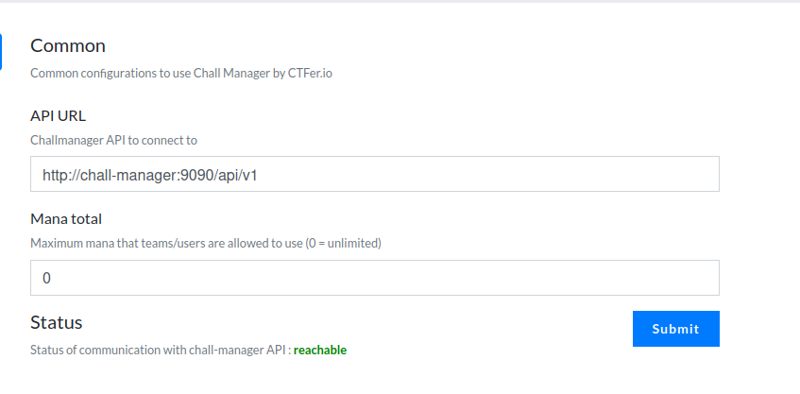
CTFd can successfully reach chall-manager
What’s next?
Congratulations! At this point, your setup is ready to use chall-manager for your CTF events.
2.1.2 - Create a challenge
Goal
In this tutorial, we will create a dynamic_iac
challenge, a new challenge type introduced by the plugin.
If you are unfamiliar with the new attributes of the dynamic_iac
challenge type, please refer to the related design.
For guidance on maintenance operations (e.g., modifying challenge attributes), please refer to the relevant guides.
For details on the Infra-as-Code scenario, consult the appropriate documentation.
Create the Challenge
For this example, we will create a challenge where each player gets their own instance. The instance will cost user 2 mana units, must be destroyed after 10 minutes without maximum due date, and using the scenario from the no-sdk examples.
Here are the basic CTFd settings:
Key | Value |
---|---|
Name | example |
Category | example |
Message | example |
Initial Value | 500 |
Decay Function | Logarithmic |
Decay | 10 |
Minimum Value | 10 |
First, configure the scope. Since we want each player to have their own instance, disable the sharing option.

Next, let the Destroy on Flag disable

Next, set the mana cost. Players will need to spend 2 mana to deploy their own instance of the challenge.

As mentioned, we want instances to be destroyed after 10 minutes of usage (600 seconds), without any due date.
Let the Until value empty, and configure de Timeout value at 600.

Then, provide the scenario archive. For this example, we’ll use demo-deploy.zip
from the no-sdk examples.
Finally, click Create to set up the challenge.
Note
When you click Create, the upload process to the chall-manager may take several seconds. Please be patient.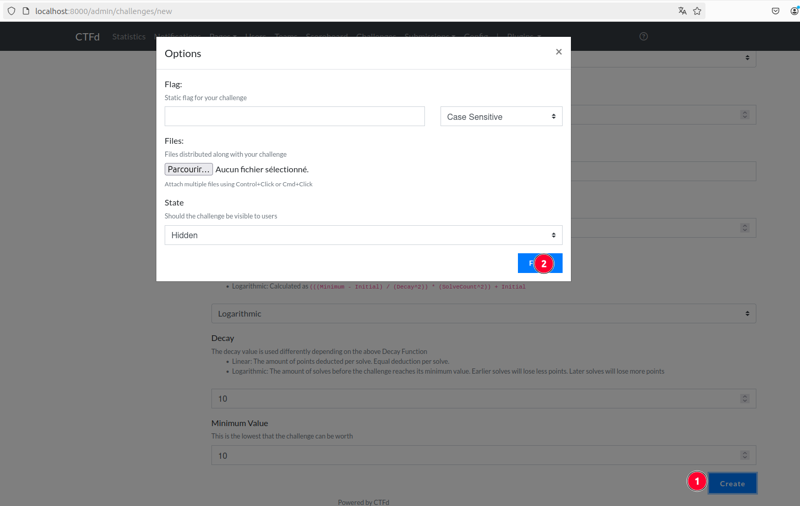
What’s Next?
Congratulations! Your CTF installation is now configured to use the chall-manager for this challenge.
2.1.3 - Play the challenge
Goal
In this tutorial we will see all actions a user has access to in order to control its instances.
Prerequisites
At this step, we assume that you are a CTF player, the infrastructure is already configured and you understand the key concepts, if not please refer to associated design.
Differents challenges mode
You can combine the values Until and Timeout to have 4 modes according to your needs:
Players can control all combinaisons, but each has these specificities.
Note
If the sharing option is enabled, only admins can launch the instance, see associated guide.For all challenges, the default view (instance is not booted) will display a button to launch the instance, the mana cost and the remaining mana for current user.
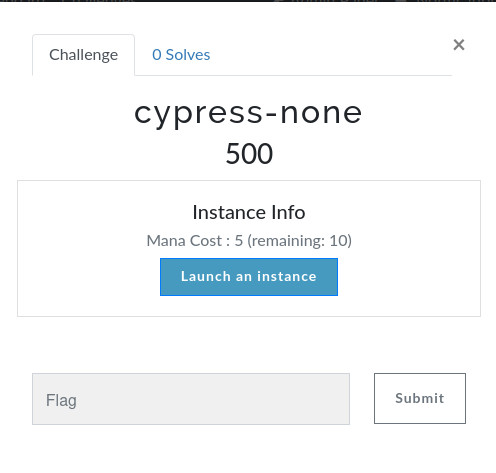
To start the instance, click on the button.
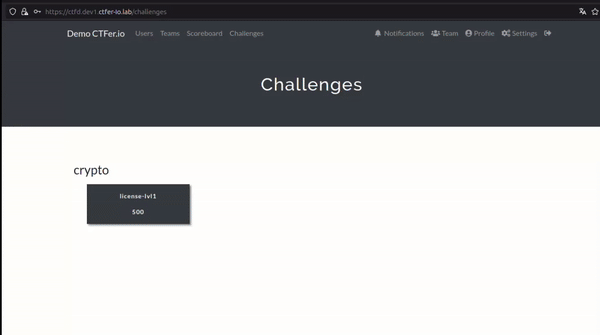
None
This mode allows you to use your instance with no duration limit. Its deployment can cost you mana, but if you want to regain it, you need to destroy the instance. If you want to reset your instance (either because it is soft-locked or you corrupted it), you can restart it.
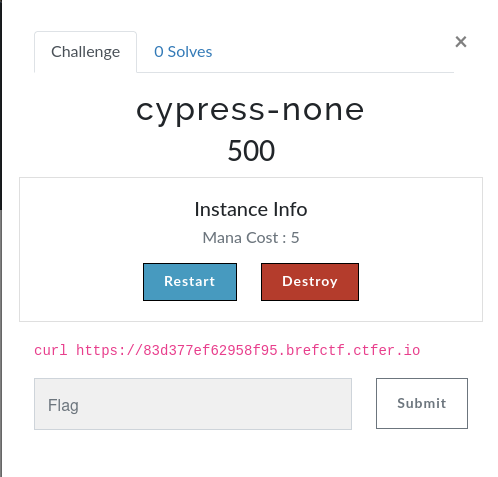
Until
This mode allows you to use the same actions as None mode, but the instance will be destroyed by the Janitor at a due date. Don’t worry, your mana will be automatically regained if the instance is janitored.
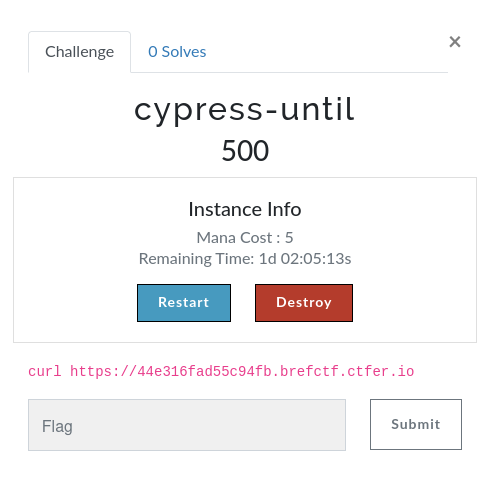
Timeout
This mode allows you to use the same actions as None and Until, but the instance will be destroyed by the Janitor n seconds after their start.
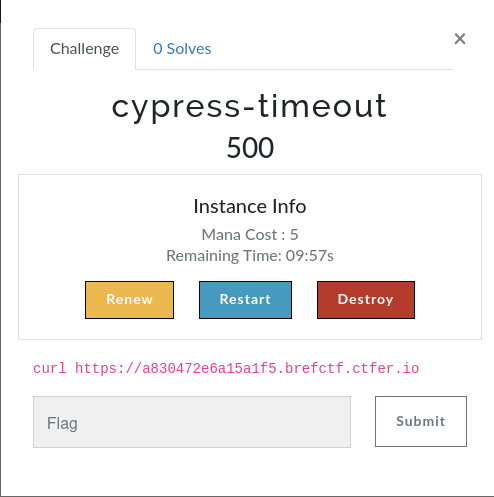
If you see that the Janitor will destroy you instance soon, you can renew the instance (reset the timer).
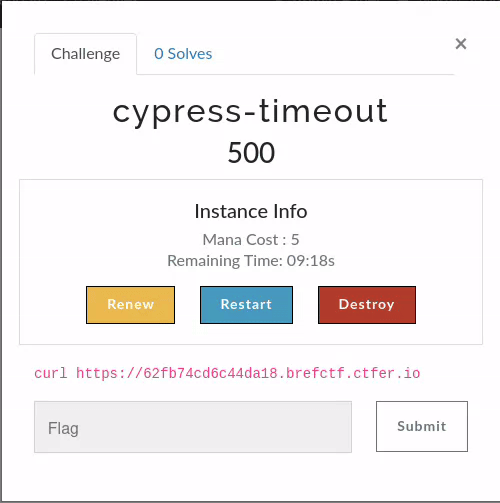
Both
With Timeout and Until, the plugin will display the timeout mode buttons, but Chall-Manager will take care of restricting or not the possibility of renewing the challenge based on the design.
What’s next ?
Congrat’s ! If you’ve made it this far, you’ll probably want to make a challenge for your event, and the plugin documentation doesn’t include explanations on how to do this, so please refer to the associated documentation.
Otherwise, you can learn more about the plugin’s design or advanced user guides here:
2.2 - Guides
2.2.1 - Settings
Goal
This guide assumes you are a CTF administrator and you understand the key concepts. Before or during your event, you may need to configure or update the plugin. At the moment, you can configure the total amount of mana for Source or and the chall-manager API URL.
Configure with environment variables
To configure the plugin at CTFd startup, you can use the next environment variables:
Variable | Default | Description |
---|---|---|
PLUGIN_SETTINGS_CM_API_URL | http://localhost:8080/api/v1 | URL of Chall-Manager API |
PLUGIN_SETTINGS_CM_MANA_TOTAL | 0 | Maximum mana that source are allowed to use |
Note
The environment variable lookup is triggered at CTFd first startup. To modify settings, you need to change it on CTFd UI.Configure in UI
To configure or perform an update, Go to CTFd Admin Panel
> Plugins
> chall-manager
> Settings
, (1) select the text input, edit it, then (2) submit form, as shown below:
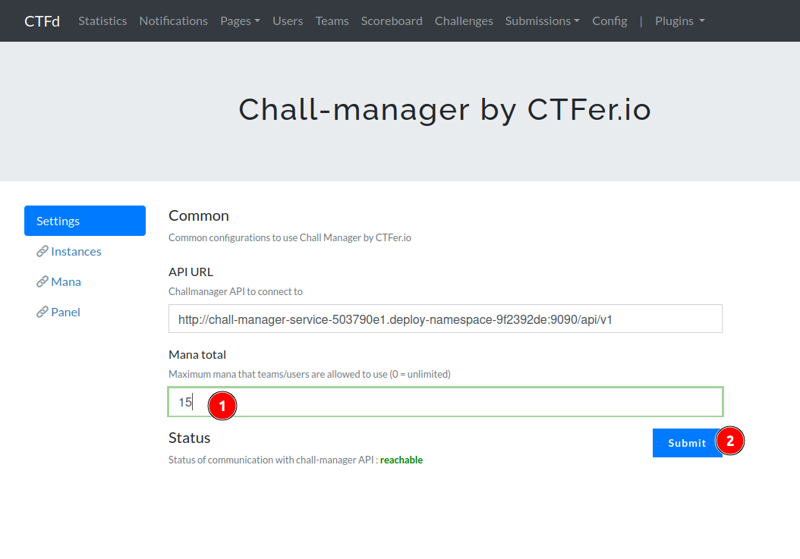
Warning
We strongly recommends you to NOT edit the chall-manager API URL during your event.Additional environment variables
The plugin can optionally use 2 commons variables to configure cache or the logging level.
Variable | Default | Description |
---|---|---|
REDIS_URL | "" | The URI to connect to a Redis server. (e.g. redis://user:password@localhost:6379) |
LOG_LEVEL | “INFO” | Enumeration in INFO, DEBUG, ERROR, WARNING |
2.2.2 - Challenge
Goal
Here, we assume that you are a CTF administrator, the infrastructure is already configured and you understand the key concepts. During or before your event you may need to change challenge attributes.
For all updates, go to the CTFd Admin Panel and edit the challenge (https://CTFD_URL/admin/challenges).
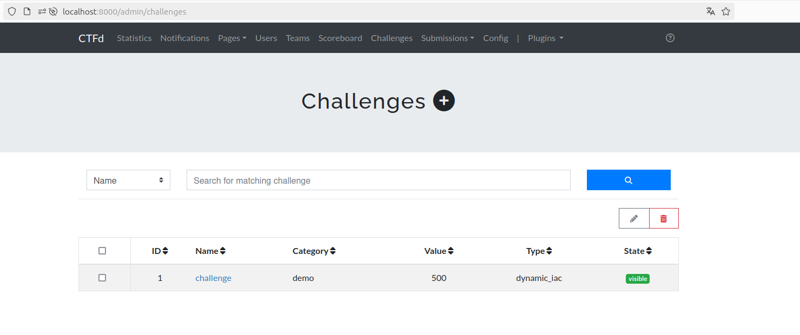
Change the scope
When you arrive on the modification page, the value displayed is the one configured at the challenge level.

By editing this value, you trigger instances destruction, see workflow belows:
flowchart LR A[Update] --> B B[Update on CTFd backend] --> C C{challenge is shared ?} C -->|True|F[Destroy all instances] C -->|False| E[Destroy the shared instance] E --> H[Send update payload to CM] F --> H
Change the destroy on flag
When you arrive on the modification page, the value displayed is the one configured at the challenge level.
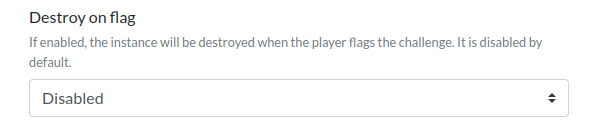
You can edit this value at any time without any impact on Chall-Manager API.
Change the mana cost
When you arrive on the modification page, the value displayed is the one configured at the challenge level.

By editing this value, you do not edit the existing coupons of this challenge. Also, you can organize sales periods.
Change Timeout
When you arrive on the modification page, the value displayed is the one configured at the challenge level.

You can change or reset this value, Chall-Manager will update all the computed until
for instances.
Change Until
When you arrive on the modification page, the value displayed is the one configured at the challenge level.
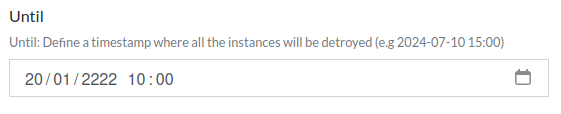
You can change or reset this value, Chall-Manager will update all the computed until
for instances.
Change the scenario
When you arrive on the modification page, you can download the current scenario archive.
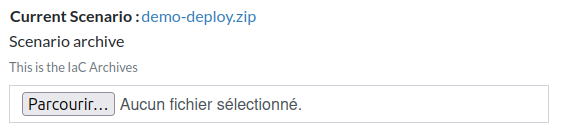
By editing this value, you need to provide an update strategy.
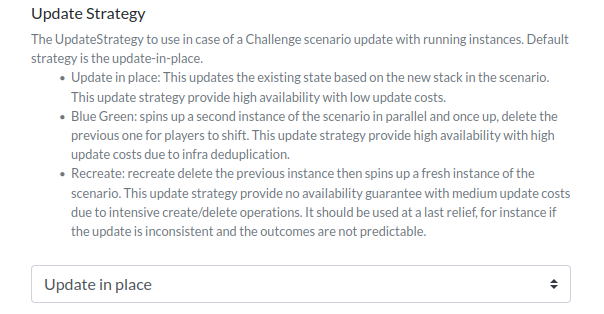
The update can be long, depends on the update gap and the strategy.
2.2.3 - Panel
Goal
Here, we assume that you are a CTF administrator, the infrastructure is already configured and you understand the key concepts.
During your event you may need to deploy instances for users we will see several use cases. If mana is enabled, 1 coupon will be created for each user.
Note
Even if users don’t have the required mana, the instance will be created here.Deploy instances for users
There may be several reasons why administrators create instances for users. Here, we’ll imagine that the CTF hasn’t started yet, that it’s a CTF in team mode and that 10 teams are expected. For the 10 teams, we want to deploy 1 instance of a challenge.
Go to CTFd Admin Panel
> Plugins
> chall-manager
> Panel
such as:
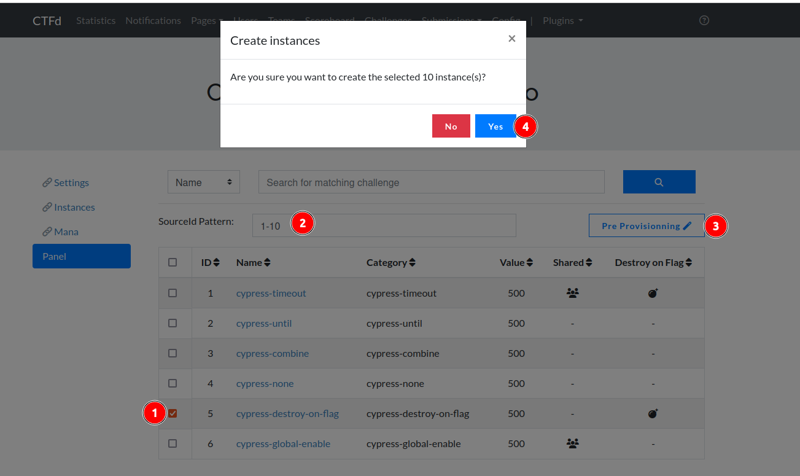
By clicking on the button, you will send several requests to CTFd. To make the user experience easier, we add a progress bar.
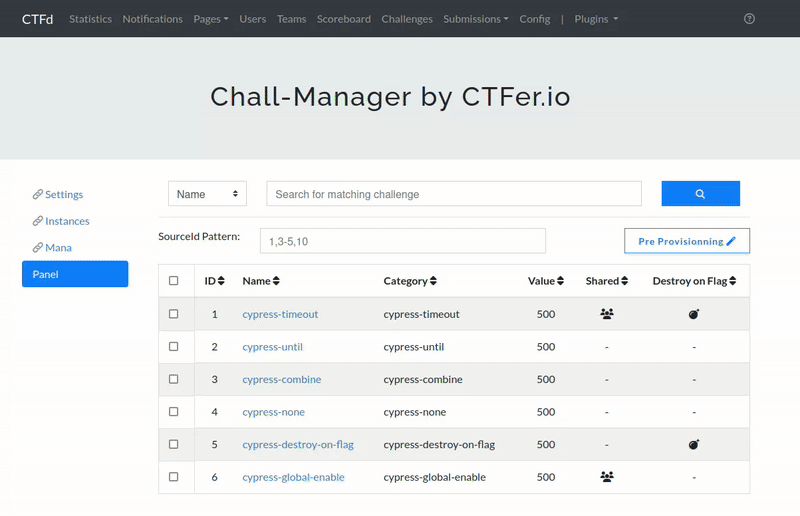
Once the progress bar is done (this mean instances are created on chall-manager), you can go the instances monitoring panel.
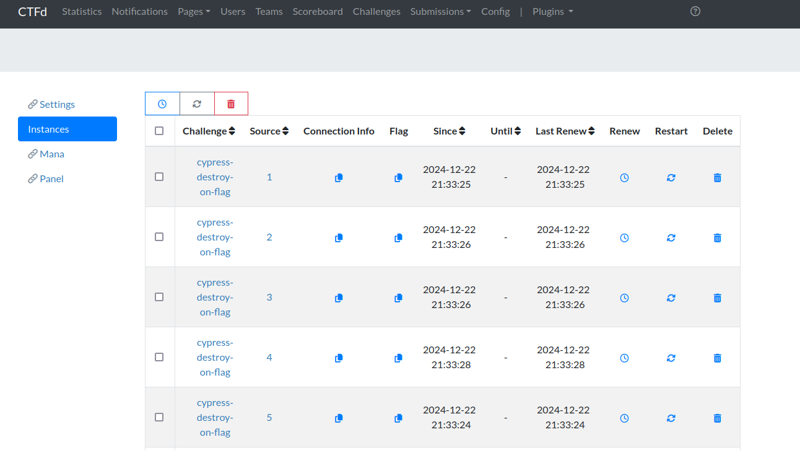
You can use this method during your event to deploy a hidden challenge (deploy instances before the challenge is release) for instance.
Deploy a shared instance
Here, we’ll imagine that you did configure a challenge with the sharing option enabled. For a shared challenge, you must put the pattern at 0, or leave it empty.
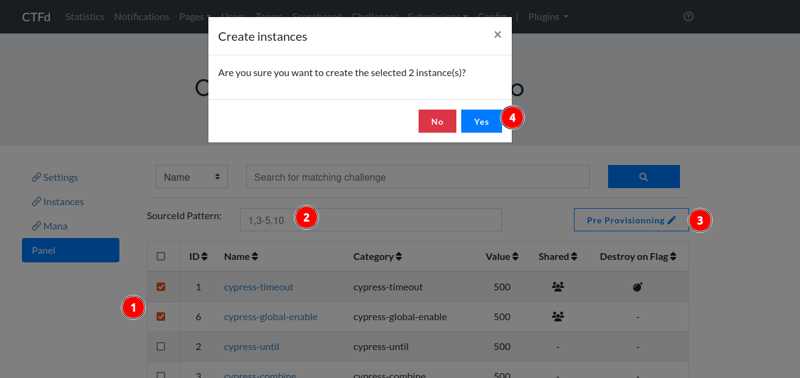
Like the previous use case, you will get a progress bar while the instance are getting deployed.
2.2.4 - Instances
Goal
This guide assumes you are a CTF administrator with a properly configured infrastructure and an understanding of key concepts.
During your event, you may need to monitor or manage instances associated with Sources.
How to do it
Monitoring
To monitor instances, go to the plugin settings in the CTFd Admin Panel
> Plugins
> chall-manager
> Instances
section.
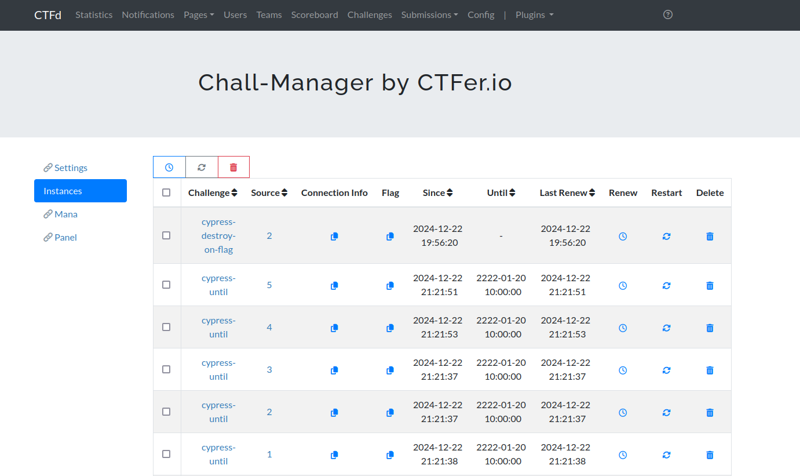
Administration
You can Renew , Restart or Destroy an individual instance by clicking the corresponding button in the instance row.
To perform actions on multiple instances, (1) select the instances using the checkboxes, (2) click the associated button, (3) then confirm your choice, as shown below:
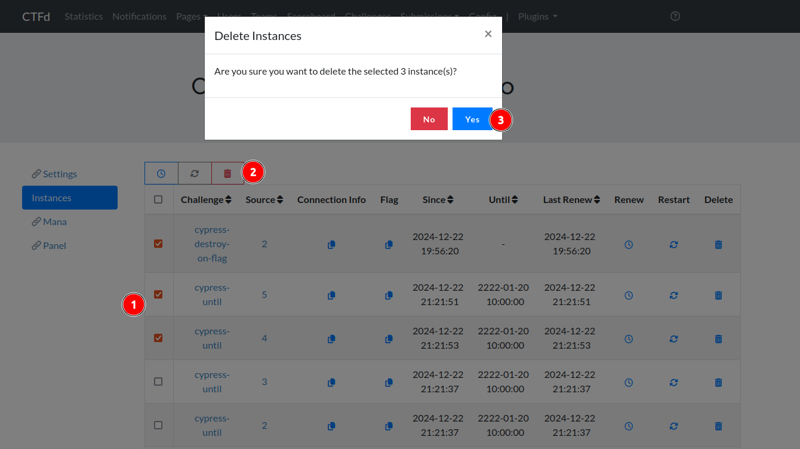
2.2.5 - Mana
Goal
Here, we assume that you are a CTF administrator, the infrastructure is already configured and you understand the key concepts.
During your event you may need to monitor the consumed mana for Source.
How to do it
Access the plugin settings configuration in the CTFd Admin Panel
> Plugins
> chall-manager
> Mana
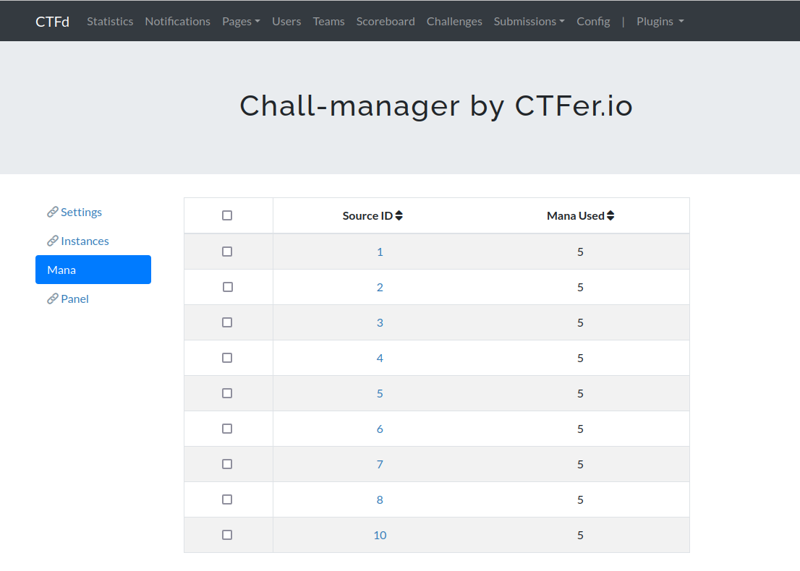
2.3 - Design
2.3.1 - Architecture
Concept
As explained in the chall-manager documentation, we avoid exposing its API to prevent the risk of direct resource manipulation by players.
CTFd inherently provides functionalities like authentication, team management, scoring, and flag handling. By adding our plugin, CTFd can serve as both a challenge management platform for administrators and a request manager that acts as a proxy with user authentication, mana limitations, and more.
Overview
The basic architecture is straightforward: we have created new API endpoints for both administrators and users. These endpoints mainly handle CRUD operations on challenge instances.
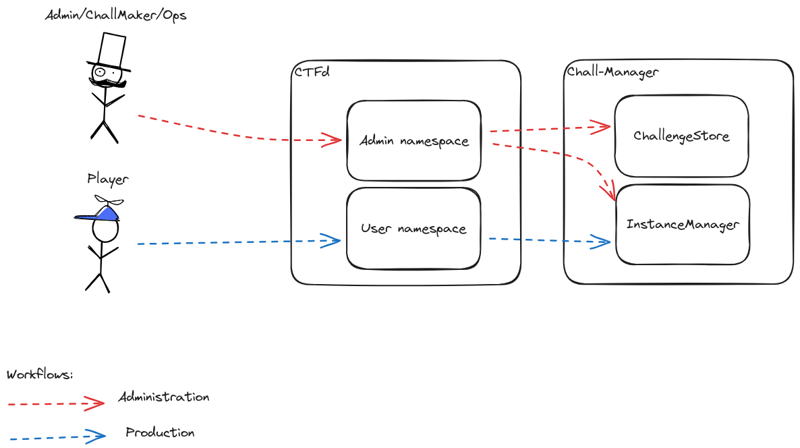
API
AdminInstance
This endpoint allows administrators to perform CRUD operations on challengeId for a specified sourceId. Essentially, this endpoint forwards requests to the chall-manager for processing.
UserInstance
Unlike the AdminInstance endpoint, this one does not accept sourceId as a parameter. Instead, it automatically identifies the source issuing the request and checks mana availability before forwarding the request to the chall-manager.
UserMana
This endpoint handles GET requests to display the remaining mana of the source issuing the request.
Detailed Overview
The following diagram provides a more detailed view of how your browser interacts with the API endpoints and how these endpoints are mapped to the corresponding chall-manager endpoints.
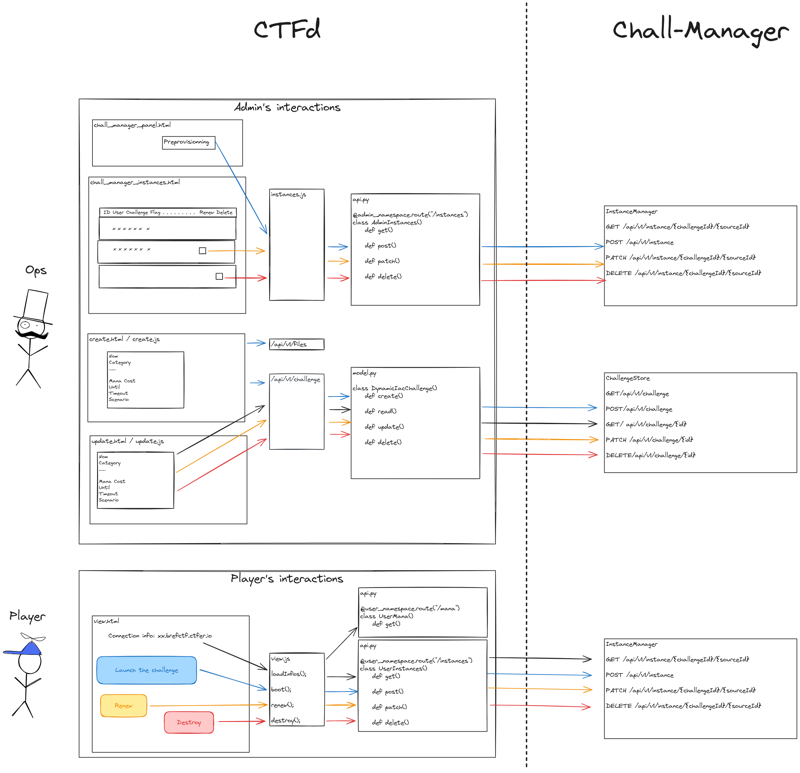
2.3.2 - Challenge
Overview
The plugin introduces a new challenge type called dynamic_iac
, allowing the deployment of instances per user or team (referred to as a source). It works seamlessly with both user modes defined by CTFd (individual users or teams).
What’s new about this challenge type?
To get started, navigate to the challenge creation panel in CTFd and select the dynamic_iac
challenge type.
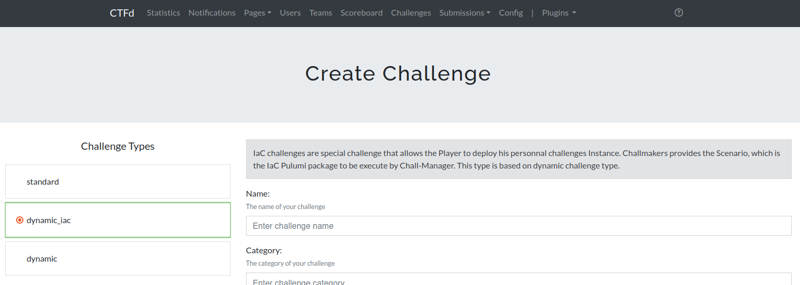
Here’s what the plugin adds:
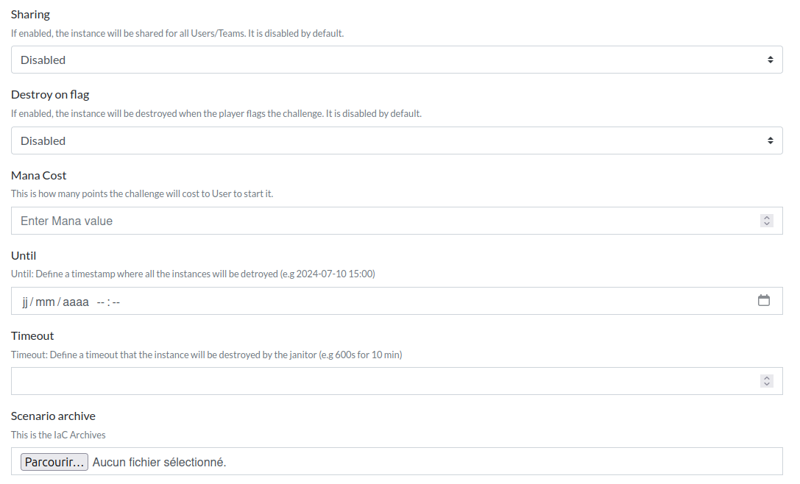
Sharing
The sharing or shared is a boolean setting that allows a single instance to be shared among all players.
For instance, in the following setup, challenges 1, 2, and 3 have the sharing enabled, while challenge 4 does not:
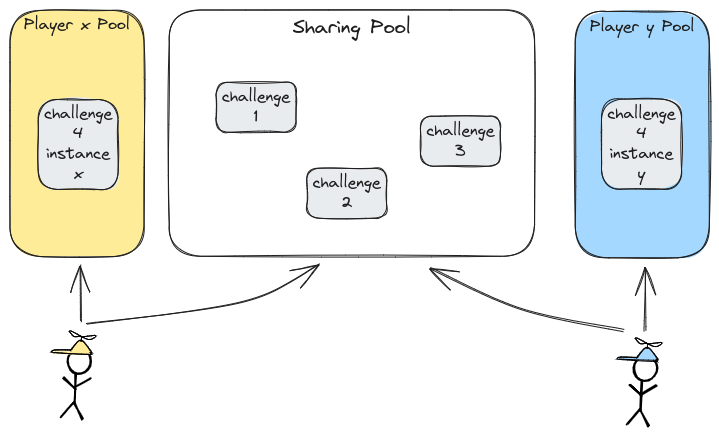
In this scenario, player X (yellow) and player Y (blue) will each have their own instances for challenge 4, but will share the same instance for challenges 1, 2, and 3. We recommend enabling this feature for static, stateless applications (e.g., websites).
Destroy on flag
Challenges with the destroy-on-flag option will automatically destroy the instance when the player submits the correct flag.
Please note: enabling this option will slow down CTFd’s response time when the correct flag is submitted due to instance destruction. We recommend that you only activate this option if you don’t want to use mana.
Mana Cost
The mana cost is an integer representing the price users must pay in mana to deploy their own instance. Mana is refunded when the instance is destroyed. This system helps control the impact users have on the platform. For more details, see how mana works.
Until
The until setting allows you to specify a date and time at which instances will be automatically destroyed by the Janitor.
Example:
As a CTF administrator running a week-long event, you want a challenge available only on the first day. Set the Janitoring Strategy to Until, and configure the end date to DD/MM/YYYY 23:59.
As a player, you can start your instance anytime before this date and destroy it whenever you like before the deadline.
Timeout
The timeout is an integer that specifies, in seconds, how long after starting an instance the Janitor will automatically destroy it.
Example:
As a CTF administrator or challenge creator, you estimate that your challenge takes about 30 minutes to solve. Set the Janitoring Strategy to Timeout and set the value to 1800 seconds (30 minutes).
As a player, once you start your instance, it will be destroyed after 30 minutes unless renewed. You can also manually destroy the instance at any time to reclaim your mana.
Tips & Tricks
You can either combine the Until and Timeout values or leave both undefined. Find more info here.Scenario Archive
The scenario is a zip archive defining the challenge deployment as a Golang Pulumi project. You can use examples or create your own using the SDK and Pulumi.
2.3.3 - Flag
Concept
Cheating is a part of competitions, especially cash-prize is involved. The player experience is particularly frustrating, so we try to minimize some of the common cases of cheating.
We’ve concentrated on the two most common cases, namely ShareFlag and FlagHolding:
- ShareFlag: get or share flags with an another team.
- FlagHolding: store flags to submit them on the very last moment.
How this work
We redefine the submit
method of the dynamic_iac
challenge to verify the generated flag against the current source.
Here’s the algorithm:
flowchart LR Submission --> A{Instance On ?} A -->|True| B{Instance I flag ?} A -->|False| Expired B -->|True| C{submission == I.flag} B -->|False| D{CTFd C flag ?} C -->|True| Correct C -->|False| D D -->|True| E{submission == C.flag} D -->|False| Incorrect E -->|True| Correct E -->|False| Incorrect %% I/O Submission Expired Correct Incorrect
On the submit
method, we get all informations of the instance on chall-manager.
- We want the instance ON to prevent FlagHolding and make sure the submitted flag is the one in the instance.
- We check if the instance define a flag (if you don’t use the flag variation on the sdk).
- If the instance define a flag, we check if the submission is correct.
- If the instance does not define a flag or if the submission is incorrect, we check if a flag is defined on CTFd (also use as fallback).
- Now, it is the classic behavior of CTFd.
Conclusion
Addressing the shareflag issue is crucial to maintaining fairness in CTF competitions. By redefining the submission process with methods like the dynamic_iac challenge, we ensure that only legitimate efforts are rewarded, preserving the true spirit and integrity of the competition.
FAQ
Why don’t we use the CTFd Flag system for generated flag ?
That’s simple: if we generate the flag on CTFd, each team can submit any generated flag, which doesn’t address the shareflag issue.
Why must the instance be ON to submit?
There are two main reasons for this requirement:
- To prevent ShareFlag: the instance must be up and running to retrieve the generated flag from chall-manager, ensuring flags are unique and not shared between participants.
- To prevent FlagHolding: keeping the instance active forces players to consume their mana when submitting flags, encouraging continuous participation in the competition. Additionnaly, the generated flag is valid for a running instance and will be regenerated in case of recreation.
Why the CTFd Flag is consider as “fallback” ?
As we said before, the CTFd Flag system allows users to submit the same flag. We use this system to prevent connection error or latency with chall-manager or if the generated flag is invalid for synthax error (we choose the extended ASCII so it should not happen).
2.3.4 - Source
Concept
As explained here, the Source refers to the team or user that initiates a request. This abstraction allows compatibility with CTFd operating in either “users” or “teams” mode, making the plugin versatile and usable in both modes.
To enable sharing across all users, the sourceId
is set to 0
. The table below summarizes the different scenarios:
user_mode | shared | sourceId |
---|---|---|
users | FALSE | current_user.id |
teams | FALSE | current_user.team_id |
users | TRUE | 0 |
teams | TRUE | 0 |
Example workflow
Instance creation
Here an example of sourceId usage in the instance creation process:
flowchart LR A[Launch] --> B{CTFd mode} B -->|users| C[sourceId = user.id] B -->|teams| D[sourceId = user.team_id] D --> E{challenge.shared ?} C --> E E -->|True| F[sourceId = 0] E -->|False| End1 F --> End1 End1(((1)))
The rest of the workflows is detailed in mana section.
2.3.5 - Mana
Concept
As a CTF administrator, it’s important to manage infrastructure resources such as VMs, containers, or Kubernetes Pods effectively. To avoid overloading the infrastructure by deploying excessive instances, we introduced a “mana” system that assigns a cost to each challenge.
For instance, consider these three challenges:
- challenge1: a static web server in the web category;
- challenge2: a Unix shell challenge in the pwn category;
- challenge3: a Windows server VM in the forensic category.
Challenge3 demands more resources than Challenge2, and Challenge2 requires more than Challenge1.
Suppose each team has a mana limit of 5, with the following costs assigned:
Name | Cost |
---|---|
challenge1 | 1 |
challenge2 | 2 |
challenge3 | 5 |
In this scenario, teams can deploy instances for Challenge1 and Challenge2 simultaneously but must remove both to deploy Challenge3.
Example:
- Team 1: Focused on pwn challenges, will likely prioritize deploying Challenge2.
- Team 2: Specializes in Windows forensics, making Challenge3 their main priority.
This system helps administrators control the use of resources on CTF infrastructures.
Combined with the flag variation engine feature of chall-manager, mana also helps minimize Flag Holding. To progress, players must submit flags and destroy instances to reclaim mana. If players choose to hoard flags until the last moment, mana limits their ability to continue, adding a strategic element to the competition.
How it works
Mana Total
To set the total mana available to users, go to the Settings section of the plugin and adjust the “Mana Total” field. By default, this feature is disabled with a value of 0. The total mana can be modified during the event without any impact on existing configurations.
Mana Cost
Each dynamic_iac
challenge is assigned a mana cost, which can be set to 0 if no cost is desired. Changes to mana costs can be made during the event, but previously issued coupons will retain the cost at the time of their creation.
Coupons
When a user requests an instance, a coupon is generated as long as they have enough mana. Administrators can bypass restrictions and deploy instances directly through the admin panel, creating coupons without limits. Each coupon stores the sourceId, challengeId, and challengeManaCost at the time of creation.
Example workflow
Instance creation
Here an example of the usage of mana and the coupon creation while the instance create process:
flowchart LR End1(((1))) End1 --> G{Mana is enabled ?} G -->|True|H{Source can afford ?} G -->|False|I[Deploy instance on CM] H -->|True|J[Create coupon] H -->|False|End J --> I I --> K{Instance is deployed ?} K -->|True|M[End] K -->|False|N{Mana is enabled?} N -->|True|L[Destroy coupon] N -->|False|M L --> M
Detailed process:
- We check that Mana Total is greater than 0.
- If mana is enable, we check that the Source can afford the current Mana Cost.
- If Source cannot afford the instance, the process end.
- If Source can afford, we create a coupon.
- While the coupon is created, or the mana feature is disable, we create the instance on chall-manager.
- We check that the instance is running on Chall-Manager.
- If it’s running correctly, we end the process.
- If not, we destroy the coupon if exists, then end process with an error.
Synchronicity
Due to the vital role of mana, we have to ensure its consistency: elseway it could be possible to brute-force the use of Chall-Manager to either run all possible challenges thus bypassing restrictions.
To provide this strict consistency, we implemented a Writer-preference Reader-Writer problem based upon Courtois et al. (1971) work. We model the problem such as administration tasks are Writers and players Readers, hence administration has priority over players. For scalability, we needed a distributed lock system, and due to CTFd’s use of Redis, we choosed to reuse it.
FAQ
Why aren’t previous coupon prices updated?
We modeled the mana mechanism on a pricing strategy similar to retail: prices can fluctuate during promotions, and when an item is returned, the customer receives the price paid at purchase. This approach allows CTF administrators to adjust challenge costs dynamically while maintaining consistency.
Why can administrators bypass the mana check?
During CTF events, administrators might need to deploy instances for users who have exhausted their mana. While coupons will still be generated and mana consumed, administrators can set the cost to 0 at the challenge level to waive the charge if needed.
2.3.6 - Testing
Purpose
Developing in an Open Source ecosystem is good, being active in responding to problems is better, detecting problems before they affect the end-user is the ideal goal!
Following this philosophy, we put a lot of effort into CI testing, mainly using Cypress, and analyze code security via CodeQL.
GitHub Actions
From a technology point of view, we use GitHub Actions whenever possible to automate our tests.
Security
To ensure ongoing security, we enable advanced security analysis on the repository and conduct periodic scans with CodeQL on each pull request.
Testing
Our tests are systematically executed on each push and pull request, following a two-stage process.
The first stage involves Cypress for plugin integration, where we validate correct test cases to ensure comprehensive coverage. The second stage is executed to check for potential error with invalid cases. This stage runs after the Cypress tests to save time and due to technical constraints, such as the creation of challenges (preconditions).
To detect potential divergences with CTFd mode, we execute the tests in mode: users
and mode: teams
.
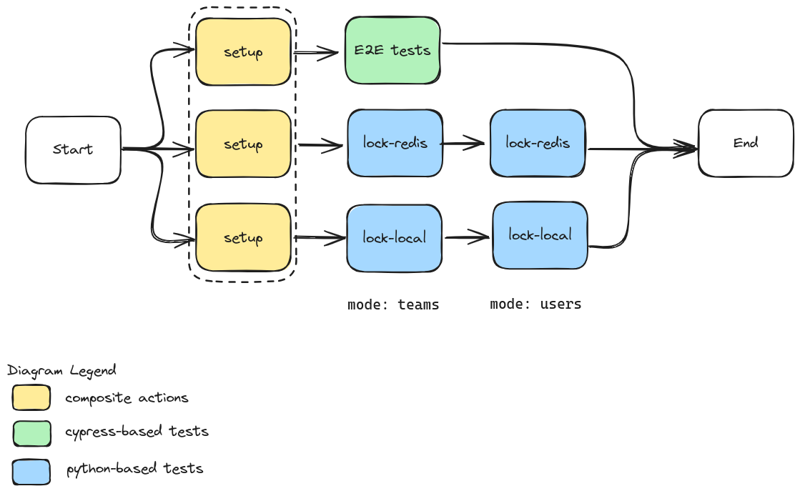